21 Jan 2025
Kubernetes Blog
Spotlight on SIG Architecture: Enhancements
This is the fourth interview of a SIG Architecture Spotlight series that will cover the different subprojects, and we will be covering SIG Architecture: Enhancements.
In this SIG Architecture spotlight we talked with Kirsten Garrison, lead of the Enhancements subproject.
The Enhancements subproject
Frederico (FSM): Hi Kirsten, very happy to have the opportunity to talk about the Enhancements subproject. Let's start with some quick information about yourself and your role.
Kirsten Garrison (KG): I'm a lead of the Enhancements subproject of SIG-Architecture and currently work at Google. I first got involved by contributing to the service-catalog project with the help of Carolyn Van Slyck. With time, I joined the Release team, eventually becoming the Enhancements Lead and a Release Lead shadow. While on the release team, I worked on some ideas to make the process better for the SIGs and Enhancements team (the opt-in process) based on my team's experiences. Eventually, I started attending Subproject meetings and contributing to the Subproject's work.
FSM: You mentioned the Enhancements subproject: how would you describe its main goals and areas of intervention?
KG: The Enhancements Subproject primarily concerns itself with the Kubernetes Enhancement Proposal (KEP for short)-the "design" documents required for all features and significant changes to the Kubernetes project.
The KEP and its impact
FSM: The improvement of the KEP process was (and is) one in which SIG Architecture was heavily involved. Could you explain the process to those that aren't aware of it?
KG: Every release, the SIGs let the Release Team know which features they intend to work on to be put into the release. As mentioned above, the prerequisite for these changes is a KEP - a standardized design document that all authors must fill out and approve in the first weeks of the release cycle. Most features will move through 3 phases: alpha, beta and finally GA so approving a feature represents a significant commitment for the SIG.
The KEP serves as the full source of truth of a feature. The KEP template has different requirements based on what stage a feature is in, but it generally requires a detailed discussion of the design and the impact as well as providing artifacts of stability and performance. The KEP takes quite a bit of iterative work between authors, SIG reviewers, api review team and the Production Readiness Review team1 before it is approved. Each set of reviewers is looking to make sure that the proposal meets their standards in order to have a stable and performant Kubernetes release. Only after all approvals are secured, can an author go forth and merge their feature in the Kubernetes code base.
FSM: I see, quite a bit of additional structure was added. Looking back, what were the most significant improvements of that approach?
KG: In general, I think that the improvements with the most impact had to do with focusing on the core intent of the KEP. KEPs exist not just to memorialize designs, but provide a structured way to discuss and come to an agreement about different facets of the change. At the core of the KEP process is communication and consideration.
To that end, some of the significant changes revolve around a more detailed and accessible KEP template. A significant amount of work was put in over time to get the k/enhancements repo into its current form -- a directory structure organized by SIG with the contours of the modern KEP template (with Proposal/Motivation/Design Details subsections). We might take that basic structure for granted today, but it really represents the work of many people trying to get the foundation of this process in place over time.
As Kubernetes matures, we've needed to think about more than just the end goal of getting a single feature merged. We need to think about things like: stability, performance, setting and meeting user expectations. And as we've thought about those things the template has grown more detailed. The addition of the Production Readiness Review was major as well as the enhanced testing requirements (varying at different stages of a KEP's lifecycle).
Current areas of focus
FSM: Speaking of maturing, we've recently released Kubernetes v1.31, and work on v1.32 has started. Are there any areas that the Enhancements sub-project is currently addressing that might change the way things are done?
KG: We're currently working on two things:
- Creating a Process KEP template. Sometimes people want to harness the KEP process for significant changes that are more process oriented rather than feature oriented. We want to support this because memorializing changes is important and giving people a better tool to do so will only encourage more discussion and transparency.
- KEP versioning. While our template changes aim to be as non-disruptive as possible, we believe that it will be easier to track and communicate those changes to the community better with a versioned KEP template and the policies that go alongside such versioning.
Both features will take some time to get right and fully roll out (just like a KEP feature) but we believe that they will both provide improvements that will benefit the community at large.
FSM: You mentioned improvements: I remember when project boards for Enhancement tracking were introduced in recent releases, to great effect and unanimous applause from release team members. Was this a particular area of focus for the subproject?
KG: The Subproject provided support to the Release Team's Enhancement team in the migration away from using the spreadsheet to a project board. The collection and tracking of enhancements has always been a logistical challenge. During my time on the Release Team, I helped with the transition to an opt-in system of enhancements, whereby the SIG leads "opt-in" KEPs for release tracking. This helped to enhance communication between authors and SIGs before any significant work was undertaken on a KEP and removed toil from the Enhancements team. This change used the existing tools to avoid introducing too many changes at once to the community. Later, the Release Team approached the Subproject with an idea of leveraging GitHub Project Boards to further improve the collection process. This was to be a move away from the use of complicated spreadsheets to using repo-native labels on k/enhancement issues and project boards.
FSM: That surely adds an impact on simplifying the workflow...
KG: Removing sources of friction and promoting clear communication is very important to the Enhancements Subproject. At the same time, it's important to give careful consideration to decisions that impact the community as a whole. We want to make sure that changes are balanced to give an upside and while not causing any regressions and pain in the rollout. We supported the Release Team in ideation as well as through the actual migration to the project boards. It was a great success and exciting to see the team make high impact changes that helped everyone involved in the KEP process!
Getting involved
FSM: For those reading that might be curious and interested in helping, how would you describe the required skills for participating in the sub-project?
KG: Familiarity with KEPs either via experience or taking time to look through the kubernetes/enhancements repo is helpful. All are welcome to participate if interested - we can take it from there.
FSM: Excellent! Many thanks for your time and insight -- any final comments you would like to share with our readers?
KG: The Enhancements process is one of the most important parts of Kubernetes and requires enormous amounts of coordination and collaboration of people and teams across the project to make it successful. I'm thankful and inspired by everyone's continued hard work and dedication to making the project great. This is truly a wonderful community.
-
For more information, check the Production Readiness Review spotlight interview in this series. ↩︎
21 Jan 2025 12:00am GMT
18 Dec 2024
Kubernetes Blog
Kubernetes 1.32: Moving Volume Group Snapshots to Beta
Volume group snapshots were introduced as an Alpha feature with the Kubernetes 1.27 release. The recent release of Kubernetes v1.32 moved that support to beta. The support for volume group snapshots relies on a set of extension APIs for group snapshots. These APIs allow users to take crash consistent snapshots for a set of volumes. Behind the scenes, Kubernetes uses a label selector to group multiple PersistentVolumeClaims for snapshotting. A key aim is to allow you restore that set of snapshots to new volumes and recover your workload based on a crash consistent recovery point.
This new feature is only supported for CSI volume drivers.
An overview of volume group snapshots
Some storage systems provide the ability to create a crash consistent snapshot of multiple volumes. A group snapshot represents copies made from multiple volumes, that are taken at the same point-in-time. A group snapshot can be used either to rehydrate new volumes (pre-populated with the snapshot data) or to restore existing volumes to a previous state (represented by the snapshots).
Why add volume group snapshots to Kubernetes?
The Kubernetes volume plugin system already provides a powerful abstraction that automates the provisioning, attaching, mounting, resizing, and snapshotting of block and file storage.
Underpinning all these features is the Kubernetes goal of workload portability: Kubernetes aims to create an abstraction layer between distributed applications and underlying clusters so that applications can be agnostic to the specifics of the cluster they run on and application deployment requires no cluster specific knowledge.
There was already a VolumeSnapshot API that provides the ability to take a snapshot of a persistent volume to protect against data loss or data corruption. However, there are other snapshotting functionalities not covered by the VolumeSnapshot API.
Some storage systems support consistent group snapshots that allow a snapshot to be taken from multiple volumes at the same point-in-time to achieve write order consistency. This can be useful for applications that contain multiple volumes. For example, an application may have data stored in one volume and logs stored in another volume. If snapshots for the data volume and the logs volume are taken at different times, the application will not be consistent and will not function properly if it is restored from those snapshots when a disaster strikes.
It is true that you can quiesce the application first, take an individual snapshot from each volume that is part of the application one after the other, and then unquiesce the application after all the individual snapshots are taken. This way, you would get application consistent snapshots.
However, sometimes the application quiesce can be so time consuming that you want to do it less frequently, or it may not be possible to quiesce an application at all. For example, a user may want to run weekly backups with application quiesce and nightly backups without application quiesce but with consistent group support which provides crash consistency across all volumes in the group.
Kubernetes APIs for volume group snapshots
Kubernetes' support for volume group snapshots relies on three API kinds that are used for managing snapshots:
- VolumeGroupSnapshot
- Created by a Kubernetes user (or perhaps by your own automation) to request creation of a volume group snapshot for multiple persistent volume claims. It contains information about the volume group snapshot operation such as the timestamp when the volume group snapshot was taken and whether it is ready to use. The creation and deletion of this object represents a desire to create or delete a cluster resource (a group snapshot).
- VolumeGroupSnapshotContent
- Created by the snapshot controller for a dynamically created VolumeGroupSnapshot. It contains information about the volume group snapshot including the volume group snapshot ID. This object represents a provisioned resource on the cluster (a group snapshot). The VolumeGroupSnapshotContent object binds to the VolumeGroupSnapshot for which it was created with a one-to-one mapping.
- VolumeGroupSnapshotClass
- Created by cluster administrators to describe how volume group snapshots should be created, including the driver information, the deletion policy, etc.
These three API kinds are defined as CustomResourceDefinitions (CRDs). These CRDs must be installed in a Kubernetes cluster for a CSI Driver to support volume group snapshots.
What components are needed to support volume group snapshots
Volume group snapshots are implemented in the external-snapshotter repository. Implementing volume group snapshots meant adding or changing several components:
- Added new CustomResourceDefinitions for VolumeGroupSnapshot and two supporting APIs.
- Volume group snapshot controller logic is added to the common snapshot controller.
- Adding logic to make CSI calls into the snapshotter sidecar controller.
The volume snapshot controller and CRDs are deployed once per cluster, while the sidecar is bundled with each CSI driver.
Therefore, it makes sense to deploy the volume snapshot controller and CRDs as a cluster addon.
The Kubernetes project recommends that Kubernetes distributors bundle and deploy the volume snapshot controller and CRDs as part of their Kubernetes cluster management process (independent of any CSI Driver).
What's new in Beta?
-
The VolumeGroupSnapshot feature in CSI spec moved to GA in the v1.11.0 release.
-
The snapshot validation webhook was deprecated in external-snapshotter v8.0.0 and it is now removed. Most of the validation webhook logic was added as validation rules into the CRDs. Minimum required Kubernetes version is 1.25 for these validation rules. One thing in the validation webhook not moved to CRDs is the prevention of creating multiple default volume snapshot classes and multiple default volume group snapshot classes for the same CSI driver. With the removal of the validation webhook, an error will still be raised when dynamically provisioning a VolumeSnapshot or VolumeGroupSnapshot when multiple default volume snapshot classes or multiple default volume group snapshot classes for the same CSI driver exist.
-
The
enable-volumegroup-snapshot
flag in the snapshot-controller and the CSI snapshotter sidecar has been replaced by a feature gate. Since VolumeGroupSnapshot is a new API, the feature moves to Beta but the feature gate is disabled by default. To use this feature, enable the feature gate by adding the flag--feature-gates=CSIVolumeGroupSnapshot=true
when starting the snapshot-controller and the CSI snapshotter sidecar. -
The logic to dynamically create the VolumeGroupSnapshot and its corresponding individual VolumeSnapshot and VolumeSnapshotContent objects are moved from the CSI snapshotter to the common snapshot-controller. New RBAC rules are added to the common snapshot-controller and some RBAC rules are removed from the CSI snapshotter sidecar accordingly.
How do I use Kubernetes volume group snapshots
Creating a new group snapshot with Kubernetes
Once a VolumeGroupSnapshotClass object is defined and you have volumes you want to snapshot together, you may request a new group snapshot by creating a VolumeGroupSnapshot object.
The source of the group snapshot specifies whether the underlying group snapshot should be dynamically created or if a pre-existing VolumeGroupSnapshotContent should be used.
A pre-existing VolumeGroupSnapshotContent is created by a cluster administrator. It contains the details of the real volume group snapshot on the storage system which is available for use by cluster users.
One of the following members in the source of the group snapshot must be set.
selector
- a label query over PersistentVolumeClaims that are to be grouped together for snapshotting. This selector will be used to match the label added to a PVC.volumeGroupSnapshotContentName
- specifies the name of a pre-existing VolumeGroupSnapshotContent object representing an existing volume group snapshot.
Dynamically provision a group snapshot
In the following example, there are two PVCs.
NAME STATUS VOLUME CAPACITY ACCESS MODES STORAGECLASS VOLUMEATTRIBUTESCLASS AGE
pvc-0 Bound pvc-6e1f7d34-a5c5-4548-b104-01e72c72b9f2 100Mi RWO csi-hostpath-sc <unset> 2m15s
pvc-1 Bound pvc-abc640b3-2cc1-4c56-ad0c-4f0f0e636efa 100Mi RWO csi-hostpath-sc <unset> 2m7s
Label the PVCs.
% kubectl label pvc pvc-0 group=myGroup
persistentvolumeclaim/pvc-0 labeled
% kubectl label pvc pvc-1 group=myGroup
persistentvolumeclaim/pvc-1 labeled
For dynamic provisioning, a selector must be set so that the snapshot controller can find PVCs with the matching labels to be snapshotted together.
apiVersion: groupsnapshot.storage.k8s.io/v1beta1
kind: VolumeGroupSnapshot
metadata:
name: snapshot-daily-20241217
namespace: demo-namespace
spec:
volumeGroupSnapshotClassName: csi-groupSnapclass
source:
selector:
matchLabels:
group: myGroup
In the VolumeGroupSnapshot spec, a user can specify the VolumeGroupSnapshotClass which has the information about which CSI driver should be used for creating the group snapshot. A VolumGroupSnapshotClass is required for dynamic provisioning.
apiVersion: groupsnapshot.storage.k8s.io/v1beta1
kind: VolumeGroupSnapshotClass
metadata:
name: csi-groupSnapclass
annotations:
kubernetes.io/description: "Example group snapshot class"
driver: example.csi.k8s.io
deletionPolicy: Delete
As a result of the volume group snapshot creation, a corresponding VolumeGroupSnapshotContent object will be created with a volumeGroupSnapshotHandle pointing to a resource on the storage system.
Two individual volume snapshots will be created as part of the volume group snapshot creation.
NAME READYTOUSE SOURCEPVC RESTORESIZE SNAPSHOTCONTENT AGE
snapshot-0962a745b2bf930bb385b7b50c9b08af471f1a16780726de19429dd9c94eaca0 true pvc-0 100Mi snapcontent-0962a745b2bf930bb385b7b50c9b08af471f1a16780726de19429dd9c94eaca0 16m
snapshot-da577d76bd2106c410616b346b2e72440f6ec7b12a75156263b989192b78caff true pvc-1 100Mi snapcontent-da577d76bd2106c410616b346b2e72440f6ec7b12a75156263b989192b78caff 16m
Importing an existing group snapshot with Kubernetes
To import a pre-existing volume group snapshot into Kubernetes, you must also import the corresponding individual volume snapshots.
Identify the individual volume snapshot handles, manually construct a VolumeSnapshotContent object first, then create a VolumeSnapshot object pointing to the VolumeSnapshotContent object. Repeat this for every individual volume snapshot.
Then manually create a VolumeGroupSnapshotContent object, specifying the volumeGroupSnapshotHandle and individual volumeSnapshotHandles already existing on the storage system.
apiVersion: groupsnapshot.storage.k8s.io/v1beta1
kind: VolumeGroupSnapshotContent
metadata:
name: static-group-content
spec:
deletionPolicy: Delete
driver: hostpath.csi.k8s.io
source:
groupSnapshotHandles:
volumeGroupSnapshotHandle: e8779136-a93e-11ef-9549-66940726f2fd
volumeSnapshotHandles:
- e8779147-a93e-11ef-9549-66940726f2fd
- e8783cd0-a93e-11ef-9549-66940726f2fd
volumeGroupSnapshotRef:
name: static-group-snapshot
namespace: demo-namespace
After that create a VolumeGroupSnapshot object pointing to the VolumeGroupSnapshotContent object.
apiVersion: groupsnapshot.storage.k8s.io/v1beta1
kind: VolumeGroupSnapshot
metadata:
name: static-group-snapshot
namespace: demo-namespace
spec:
source:
volumeGroupSnapshotContentName: static-group-content
How to use group snapshot for restore in Kubernetes
At restore time, the user can request a new PersistentVolumeClaim to be created from a VolumeSnapshot object that is part of a VolumeGroupSnapshot. This will trigger provisioning of a new volume that is pre-populated with data from the specified snapshot. The user should repeat this until all volumes are created from all the snapshots that are part of a group snapshot.
apiVersion: v1
kind: PersistentVolumeClaim
metadata:
name: examplepvc-restored-2024-12-17
namespace: demo-namespace
spec:
storageClassName: example-foo-nearline
dataSource:
name: snapshot-0962a745b2bf930bb385b7b50c9b08af471f1a16780726de19429dd9c94eaca0
kind: VolumeSnapshot
apiGroup: snapshot.storage.k8s.io
accessModes:
- ReadWriteOncePod
resources:
requests:
storage: 100Mi # must be enough storage to fit the existing snapshot
As a storage vendor, how do I add support for group snapshots to my CSI driver?
To implement the volume group snapshot feature, a CSI driver must:
- Implement a new group controller service.
- Implement group controller RPCs:
CreateVolumeGroupSnapshot
,DeleteVolumeGroupSnapshot
, andGetVolumeGroupSnapshot
. - Add group controller capability
CREATE_DELETE_GET_VOLUME_GROUP_SNAPSHOT
.
See the CSI spec and the Kubernetes-CSI Driver Developer Guide for more details.
As mentioned earlier, it is strongly recommended that Kubernetes distributors bundle and deploy the volume snapshot controller and CRDs as part of their Kubernetes cluster management process (independent of any CSI Driver).
As part of this recommended deployment process, the Kubernetes team provides a number of sidecar (helper) containers, including the external-snapshotter sidecar container which has been updated to support volume group snapshot.
The external-snapshotter watches the Kubernetes API server for VolumeGroupSnapshotContent objects, and triggers CreateVolumeGroupSnapshot
and DeleteVolumeGroupSnapshot
operations against a CSI endpoint.
What are the limitations?
The beta implementation of volume group snapshots for Kubernetes has the following limitations:
- Does not support reverting an existing PVC to an earlier state represented by a snapshot (only supports provisioning a new volume from a snapshot).
- No application consistency guarantees beyond any guarantees provided by the storage system (e.g. crash consistency). See this doc for more discussions on application consistency.
What's next?
Depending on feedback and adoption, the Kubernetes project plans to push the volume group snapshot implementation to general availability (GA) in a future release.
How can I learn more?
- The design spec for the volume group snapshot feature.
- The code repository for volume group snapshot APIs and controller.
- CSI documentation on the group snapshot feature.
How do I get involved?
This project, like all of Kubernetes, is the result of hard work by many contributors from diverse backgrounds working together. On behalf of SIG Storage, I would like to offer a huge thank you to the contributors who stepped up these last few quarters to help the project reach beta:
- Ben Swartzlander (bswartz)
- Cici Huang (cici37)
- Hemant Kumar (gnufied)
- James Defelice (jdef)
- Jan Šafránek (jsafrane)
- Madhu Rajanna (Madhu-1)
- Manish M Yathnalli (manishym)
- Michelle Au (msau42)
- Niels de Vos (nixpanic)
- Leonardo Cecchi (leonardoce)
- Rakshith R (Rakshith-R)
- Raunak Shah (RaunakShah)
- Saad Ali (saad-ali)
- Xing Yang (xing-yang)
- Yati Padia (yati1998)
For those interested in getting involved with the design and development of CSI or any part of the Kubernetes Storage system, join the Kubernetes Storage Special Interest Group (SIG). We always welcome new contributors.
We also hold regular Data Protection Working Group meetings. New attendees are welcome to join our discussions.
18 Dec 2024 12:00am GMT
17 Dec 2024
Kubernetes Blog
Enhancing Kubernetes API Server Efficiency with API Streaming
Managing Kubernetes clusters efficiently is critical, especially as their size is growing. A significant challenge with large clusters is the memory overhead caused by list requests.
In the existing implementation, the kube-apiserver processes list requests by assembling the entire response in-memory before transmitting any data to the client. But what if the response body is substantial, say hundreds of megabytes? Additionally, imagine a scenario where multiple list requests flood in simultaneously, perhaps after a brief network outage. While API Priority and Fairness has proven to reasonably protect kube-apiserver from CPU overload, its impact is visibly smaller for memory protection. This can be explained by the differing nature of resource consumption by a single API request - the CPU usage at any given time is capped by a constant, whereas memory, being uncompressible, can grow proportionally with the number of processed objects and is unbounded. This situation poses a genuine risk, potentially overwhelming and crashing any kube-apiserver within seconds due to out-of-memory (OOM) conditions. To better visualize the issue, let's consider the below graph.
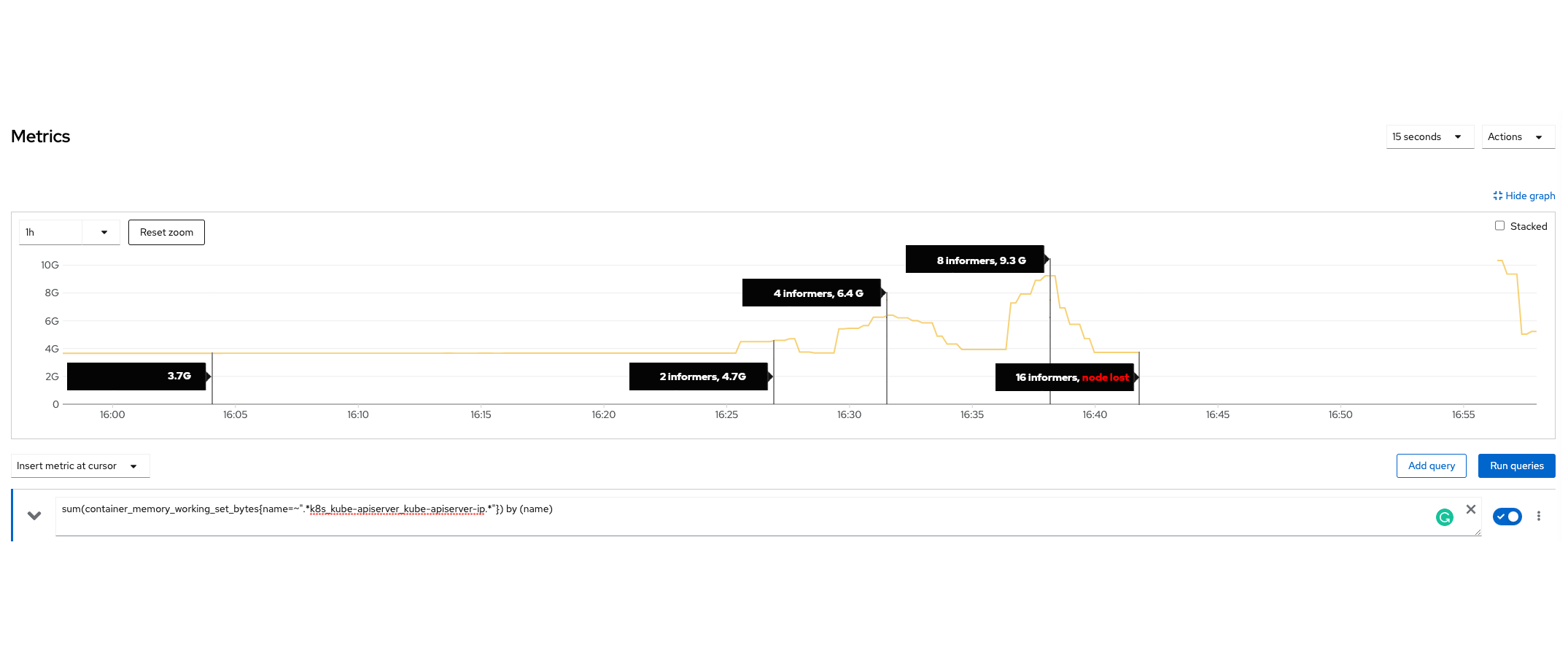
The graph shows the memory usage of a kube-apiserver during a synthetic test. (see the synthetic test section for more details). The results clearly show that increasing the number of informers significantly boosts the server's memory consumption. Notably, at approximately 16:40, the server crashed when serving only 16 informers.
Why does kube-apiserver allocate so much memory for list requests?
Our investigation revealed that this substantial memory allocation occurs because the server before sending the first byte to the client must:
- fetch data from the database,
- deserialize the data from its stored format,
- and finally construct the final response by converting and serializing the data into a client requested format
This sequence results in significant temporary memory consumption. The actual usage depends on many factors like the page size, applied filters (e.g. label selectors), query parameters, and sizes of individual objects.
Unfortunately, neither API Priority and Fairness nor Golang's garbage collection or Golang memory limits can prevent the system from exhausting memory under these conditions. The memory is allocated suddenly and rapidly, and just a few requests can quickly deplete the available memory, leading to resource exhaustion.
Depending on how the API server is run on the node, it might either be killed through OOM by the kernel when exceeding the configured memory limits during these uncontrolled spikes, or if limits are not configured it might have even worse impact on the control plane node. And worst, after the first API server failure, the same requests will likely hit another control plane node in an HA setup with probably the same impact. Potentially a situation that is hard to diagnose and hard to recover from.
Streaming list requests
Today, we're excited to announce a major improvement. With the graduation of the watch list feature to beta in Kubernetes 1.32, client-go users can opt-in (after explicitly enabling WatchListClient
feature gate) to streaming lists by switching from list to (a special kind of) watch requests.
Watch requests are served from the watch cache, an in-memory cache designed to improve scalability of read operations. By streaming each item individually instead of returning the entire collection, the new method maintains constant memory overhead. The API server is bound by the maximum allowed size of an object in etcd plus a few additional allocations. This approach drastically reduces the temporary memory usage compared to traditional list requests, ensuring a more efficient and stable system, especially in clusters with a large number of objects of a given type or large average object sizes where despite paging memory consumption used to be high.
Building on the insight gained from the synthetic test (see the synthetic test, we developed an automated performance test to systematically evaluate the impact of the watch list feature. This test replicates the same scenario, generating a large number of Secrets with a large payload, and scaling the number of informers to simulate heavy list request patterns. The automated test is executed periodically to monitor memory usage of the server with the feature enabled and disabled.
The results showed significant improvements with the watch list feature enabled. With the feature turned on, the kube-apiserver's memory consumption stabilized at approximately 2 GB. By contrast, with the feature disabled, memory usage increased to approximately 20GB, a 10x increase! These results confirm the effectiveness of the new streaming API, which reduces the temporary memory footprint.
Enabling API Streaming for your component
Upgrade to Kubernetes 1.32. Make sure your cluster uses etcd in version 3.4.31+ or 3.5.13+. Change your client software to use watch lists. If your client code is written in Golang, you'll want to enable WatchListClient
for client-go. For details on enabling that feature, read Introducing Feature Gates to Client-Go: Enhancing Flexibility and Control.
What's next?
In Kubernetes 1.32, the feature is enabled in kube-controller-manager by default despite its beta state. This will eventually be expanded to other core components like kube-scheduler or kubelet; once the feature becomes generally available, if not earlier. Other 3rd-party components are encouraged to opt-in to the feature during the beta phase, especially when they are at risk of accessing a large number of resources or kinds with potentially large object sizes.
For the time being, API Priority and Fairness assigns a reasonable small cost to list requests. This is necessary to allow enough parallelism for the average case where list requests are cheap enough. But it does not match the spiky exceptional situation of many and large objects. Once the majority of the Kubernetes ecosystem has switched to watch list, the list cost estimation can be changed to larger values without risking degraded performance in the average case, and with that increasing the protection against this kind of requests that can still hit the API server in the future.
The synthetic test
In order to reproduce the issue, we conducted a manual test to understand the impact of list requests on kube-apiserver memory usage. In the test, we created 400 Secrets, each containing 1 MB of data, and used informers to retrieve all Secrets.
The results were alarming, only 16 informers were needed to cause the test server to run out of memory and crash, demonstrating how quickly memory consumption can grow under such conditions.
Special shout out to @deads2k for his help in shaping this feature.
17 Dec 2024 12:00am GMT