14 Feb 2025
Kubernetes Blog
The Cloud Controller Manager Chicken and Egg Problem
Kubernetes 1.31 completed the largest migration in Kubernetes history, removing the in-tree cloud provider. While the component migration is now done, this leaves some additional complexity for users and installer projects (for example, kOps or Cluster API) . We will go over those additional steps and failure points and make recommendations for cluster owners. This migration was complex and some logic had to be extracted from the core components, building four new subsystems.
- Cloud controller manager (KEP-2392)
- API server network proxy (KEP-1281)
- kubelet credential provider plugins (KEP-2133)
- Storage migration to use CSI (KEP-625)
The cloud controller manager is part of the control plane. It is a critical component that replaces some functionality that existed previously in the kube-controller-manager and the kubelet.
Components of Kubernetes
One of the most critical functionalities of the cloud controller manager is the node controller, which is responsible for the initialization of the nodes.
As you can see in the following diagram, when the kubelet starts, it registers the Node object with the apiserver, Tainting the node so it can be processed first by the cloud-controller-manager. The initial Node is missing the cloud-provider specific information, like the Node Addresses and the Labels with the cloud provider specific information like the Node, Region and Instance type information.
Chicken and egg problem sequence diagram
This new initialization process adds some latency to the node readiness. Previously, the kubelet was able to initialize the node at the same time it created the node. Since the logic has moved to the cloud-controller-manager, this can cause a chicken and egg problem during the cluster bootstrapping for those Kubernetes architectures that do not deploy the controller manager as the other components of the control plane, commonly as static pods, standalone binaries or daemonsets/deployments with tolerations to the taints and using hostNetwork
(more on this below)
Examples of the dependency problem
As noted above, it is possible during bootstrapping for the cloud-controller-manager to be unschedulable and as such the cluster will not initialize properly. The following are a few concrete examples of how this problem can be expressed and the root causes for why they might occur.
These examples assume you are running your cloud-controller-manager using a Kubernetes resource (e.g. Deployment, DaemonSet, or similar) to control its lifecycle. Because these methods rely on Kubernetes to schedule the cloud-controller-manager, care must be taken to ensure it will schedule properly.
Example: Cloud controller manager not scheduling due to uninitialized taint
As noted in the Kubernetes documentation, when the kubelet is started with the command line flag --cloud-provider=external
, its corresponding Node
object will have a no schedule taint named node.cloudprovider.kubernetes.io/uninitialized
added. Because the cloud-controller-manager is responsible for removing the no schedule taint, this can create a situation where a cloud-controller-manager that is being managed by a Kubernetes resource, such as a Deployment
or DaemonSet
, may not be able to schedule.
If the cloud-controller-manager is not able to be scheduled during the initialization of the control plane, then the resulting Node
objects will all have the node.cloudprovider.kubernetes.io/uninitialized
no schedule taint. It also means that this taint will not be removed as the cloud-controller-manager is responsible for its removal. If the no schedule taint is not removed, then critical workloads, such as the container network interface controllers, will not be able to schedule, and the cluster will be left in an unhealthy state.
Example: Cloud controller manager not scheduling due to not-ready taint
The next example would be possible in situations where the container network interface (CNI) is waiting for IP address information from the cloud-controller-manager (CCM), and the CCM has not tolerated the taint which would be removed by the CNI.
The Kubernetes documentation describes the node.kubernetes.io/not-ready
taint as follows:
"The Node controller detects whether a Node is ready by monitoring its health and adds or removes this taint accordingly."
One of the conditions that can lead to a Node resource having this taint is when the container network has not yet been initialized on that node. As the cloud-controller-manager is responsible for adding the IP addresses to a Node resource, and the IP addresses are needed by the container network controllers to properly configure the container network, it is possible in some circumstances for a node to become stuck as not ready and uninitialized permanently.
This situation occurs for a similar reason as the first example, although in this case, the node.kubernetes.io/not-ready
taint is used with the no execute effect and thus will cause the cloud-controller-manager not to run on the node with the taint. If the cloud-controller-manager is not able to execute, then it will not initialize the node. It will cascade into the container network controllers not being able to run properly, and the node will end up carrying both the node.cloudprovider.kubernetes.io/uninitialized
and node.kubernetes.io/not-ready
taints, leaving the cluster in an unhealthy state.
Our Recommendations
There is no one "correct way" to run a cloud-controller-manager. The details will depend on the specific needs of the cluster administrators and users. When planning your clusters and the lifecycle of the cloud-controller-managers please consider the following guidance:
For cloud-controller-managers running in the same cluster, they are managing.
- Use host network mode, rather than the pod network: in most cases, a cloud controller manager will need to communicate with an API service endpoint associated with the infrastructure. Setting "hostNetwork" to true will ensure that the cloud controller is using the host networking instead of the container network and, as such, will have the same network access as the host operating system. It will also remove the dependency on the networking plugin. This will ensure that the cloud controller has access to the infrastructure endpoint (always check your networking configuration against your infrastructure provider's instructions).
- Use a scalable resource type.
Deployments
andDaemonSets
are useful for controlling the lifecycle of a cloud controller. They allow easy access to running multiple copies for redundancy as well as using the Kubernetes scheduling to ensure proper placement in the cluster. When using these primitives to control the lifecycle of your cloud controllers and running multiple replicas, you must remember to enable leader election, or else your controllers will collide with each other which could lead to nodes not being initialized in the cluster. - Target the controller manager containers to the control plane. There might exist other controllers which need to run outside the control plane (for example, Azure's node manager controller). Still, the controller managers themselves should be deployed to the control plane. Use a node selector or affinity stanza to direct the scheduling of cloud controllers to the control plane to ensure that they are running in a protected space. Cloud controllers are vital to adding and removing nodes to a cluster as they form a link between Kubernetes and the physical infrastructure. Running them on the control plane will help to ensure that they run with a similar priority as other core cluster controllers and that they have some separation from non-privileged user workloads.
- It is worth noting that an anti-affinity stanza to prevent cloud controllers from running on the same host is also very useful to ensure that a single node failure will not degrade the cloud controller performance.
- Ensure that the tolerations allow operation. Use tolerations on the manifest for the cloud controller container to ensure that it will schedule to the correct nodes and that it can run in situations where a node is initializing. This means that cloud controllers should tolerate the
node.cloudprovider.kubernetes.io/uninitialized
taint, and it should also tolerate any taints associated with the control plane (for example,node-role.kubernetes.io/control-plane
ornode-role.kubernetes.io/master
). It can also be useful to tolerate thenode.kubernetes.io/not-ready
taint to ensure that the cloud controller can run even when the node is not yet available for health monitoring.
For cloud-controller-managers that will not be running on the cluster they manage (for example, in a hosted control plane on a separate cluster), then the rules are much more constrained by the dependencies of the environment of the cluster running the cloud-controller-manager. The advice for running on a self-managed cluster may not be appropriate as the types of conflicts and network constraints will be different. Please consult the architecture and requirements of your topology for these scenarios.
Example
This is an example of a Kubernetes Deployment highlighting the guidance shown above. It is important to note that this is for demonstration purposes only, for production uses please consult your cloud provider's documentation.
apiVersion: apps/v1
kind: Deployment
metadata:
labels:
app.kubernetes.io/name: cloud-controller-manager
name: cloud-controller-manager
namespace: kube-system
spec:
replicas: 2
selector:
matchLabels:
app.kubernetes.io/name: cloud-controller-manager
strategy:
type: Recreate
template:
metadata:
labels:
app.kubernetes.io/name: cloud-controller-manager
annotations:
kubernetes.io/description: Cloud controller manager for my infrastructure
spec:
containers: # the container details will depend on your specific cloud controller manager
- name: cloud-controller-manager
command:
- /bin/my-infrastructure-cloud-controller-manager
- --leader-elect=true
- -v=1
image: registry/my-infrastructure-cloud-controller-manager@latest
resources:
requests:
cpu: 200m
memory: 50Mi
hostNetwork: true # these Pods are part of the control plane
nodeSelector:
node-role.kubernetes.io/control-plane: ""
affinity:
podAntiAffinity:
requiredDuringSchedulingIgnoredDuringExecution:
- topologyKey: "kubernetes.io/hostname"
labelSelector:
matchLabels:
app.kubernetes.io/name: cloud-controller-manager
tolerations:
- effect: NoSchedule
key: node-role.kubernetes.io/master
operator: Exists
- effect: NoExecute
key: node.kubernetes.io/unreachable
operator: Exists
tolerationSeconds: 120
- effect: NoExecute
key: node.kubernetes.io/not-ready
operator: Exists
tolerationSeconds: 120
- effect: NoSchedule
key: node.cloudprovider.kubernetes.io/uninitialized
operator: Exists
- effect: NoSchedule
key: node.kubernetes.io/not-ready
operator: Exists
When deciding how to deploy your cloud controller manager it is worth noting that cluster-proportional, or resource-based, pod autoscaling is not recommended. Running multiple replicas of a cloud controller manager is good practice for ensuring high-availability and redundancy, but does not contribute to better performance. In general, only a single instance of a cloud controller manager will be reconciling a cluster at any given time.
14 Feb 2025 12:00am GMT
21 Jan 2025
Kubernetes Blog
Spotlight on SIG Architecture: Enhancements
This is the fourth interview of a SIG Architecture Spotlight series that will cover the different subprojects, and we will be covering SIG Architecture: Enhancements.
In this SIG Architecture spotlight we talked with Kirsten Garrison, lead of the Enhancements subproject.
The Enhancements subproject
Frederico (FSM): Hi Kirsten, very happy to have the opportunity to talk about the Enhancements subproject. Let's start with some quick information about yourself and your role.
Kirsten Garrison (KG): I'm a lead of the Enhancements subproject of SIG-Architecture and currently work at Google. I first got involved by contributing to the service-catalog project with the help of Carolyn Van Slyck. With time, I joined the Release team, eventually becoming the Enhancements Lead and a Release Lead shadow. While on the release team, I worked on some ideas to make the process better for the SIGs and Enhancements team (the opt-in process) based on my team's experiences. Eventually, I started attending Subproject meetings and contributing to the Subproject's work.
FSM: You mentioned the Enhancements subproject: how would you describe its main goals and areas of intervention?
KG: The Enhancements Subproject primarily concerns itself with the Kubernetes Enhancement Proposal (KEP for short)-the "design" documents required for all features and significant changes to the Kubernetes project.
The KEP and its impact
FSM: The improvement of the KEP process was (and is) one in which SIG Architecture was heavily involved. Could you explain the process to those that aren't aware of it?
KG: Every release, the SIGs let the Release Team know which features they intend to work on to be put into the release. As mentioned above, the prerequisite for these changes is a KEP - a standardized design document that all authors must fill out and approve in the first weeks of the release cycle. Most features will move through 3 phases: alpha, beta and finally GA so approving a feature represents a significant commitment for the SIG.
The KEP serves as the full source of truth of a feature. The KEP template has different requirements based on what stage a feature is in, but it generally requires a detailed discussion of the design and the impact as well as providing artifacts of stability and performance. The KEP takes quite a bit of iterative work between authors, SIG reviewers, api review team and the Production Readiness Review team1 before it is approved. Each set of reviewers is looking to make sure that the proposal meets their standards in order to have a stable and performant Kubernetes release. Only after all approvals are secured, can an author go forth and merge their feature in the Kubernetes code base.
FSM: I see, quite a bit of additional structure was added. Looking back, what were the most significant improvements of that approach?
KG: In general, I think that the improvements with the most impact had to do with focusing on the core intent of the KEP. KEPs exist not just to memorialize designs, but provide a structured way to discuss and come to an agreement about different facets of the change. At the core of the KEP process is communication and consideration.
To that end, some of the significant changes revolve around a more detailed and accessible KEP template. A significant amount of work was put in over time to get the k/enhancements repo into its current form -- a directory structure organized by SIG with the contours of the modern KEP template (with Proposal/Motivation/Design Details subsections). We might take that basic structure for granted today, but it really represents the work of many people trying to get the foundation of this process in place over time.
As Kubernetes matures, we've needed to think about more than just the end goal of getting a single feature merged. We need to think about things like: stability, performance, setting and meeting user expectations. And as we've thought about those things the template has grown more detailed. The addition of the Production Readiness Review was major as well as the enhanced testing requirements (varying at different stages of a KEP's lifecycle).
Current areas of focus
FSM: Speaking of maturing, we've recently released Kubernetes v1.31, and work on v1.32 has started. Are there any areas that the Enhancements sub-project is currently addressing that might change the way things are done?
KG: We're currently working on two things:
- Creating a Process KEP template. Sometimes people want to harness the KEP process for significant changes that are more process oriented rather than feature oriented. We want to support this because memorializing changes is important and giving people a better tool to do so will only encourage more discussion and transparency.
- KEP versioning. While our template changes aim to be as non-disruptive as possible, we believe that it will be easier to track and communicate those changes to the community better with a versioned KEP template and the policies that go alongside such versioning.
Both features will take some time to get right and fully roll out (just like a KEP feature) but we believe that they will both provide improvements that will benefit the community at large.
FSM: You mentioned improvements: I remember when project boards for Enhancement tracking were introduced in recent releases, to great effect and unanimous applause from release team members. Was this a particular area of focus for the subproject?
KG: The Subproject provided support to the Release Team's Enhancement team in the migration away from using the spreadsheet to a project board. The collection and tracking of enhancements has always been a logistical challenge. During my time on the Release Team, I helped with the transition to an opt-in system of enhancements, whereby the SIG leads "opt-in" KEPs for release tracking. This helped to enhance communication between authors and SIGs before any significant work was undertaken on a KEP and removed toil from the Enhancements team. This change used the existing tools to avoid introducing too many changes at once to the community. Later, the Release Team approached the Subproject with an idea of leveraging GitHub Project Boards to further improve the collection process. This was to be a move away from the use of complicated spreadsheets to using repo-native labels on k/enhancement issues and project boards.
FSM: That surely adds an impact on simplifying the workflow...
KG: Removing sources of friction and promoting clear communication is very important to the Enhancements Subproject. At the same time, it's important to give careful consideration to decisions that impact the community as a whole. We want to make sure that changes are balanced to give an upside and while not causing any regressions and pain in the rollout. We supported the Release Team in ideation as well as through the actual migration to the project boards. It was a great success and exciting to see the team make high impact changes that helped everyone involved in the KEP process!
Getting involved
FSM: For those reading that might be curious and interested in helping, how would you describe the required skills for participating in the sub-project?
KG: Familiarity with KEPs either via experience or taking time to look through the kubernetes/enhancements repo is helpful. All are welcome to participate if interested - we can take it from there.
FSM: Excellent! Many thanks for your time and insight -- any final comments you would like to share with our readers?
KG: The Enhancements process is one of the most important parts of Kubernetes and requires enormous amounts of coordination and collaboration of people and teams across the project to make it successful. I'm thankful and inspired by everyone's continued hard work and dedication to making the project great. This is truly a wonderful community.
-
For more information, check the Production Readiness Review spotlight interview in this series. ↩︎
21 Jan 2025 12:00am GMT
18 Dec 2024
Kubernetes Blog
Kubernetes 1.32: Moving Volume Group Snapshots to Beta
Volume group snapshots were introduced as an Alpha feature with the Kubernetes 1.27 release. The recent release of Kubernetes v1.32 moved that support to beta. The support for volume group snapshots relies on a set of extension APIs for group snapshots. These APIs allow users to take crash consistent snapshots for a set of volumes. Behind the scenes, Kubernetes uses a label selector to group multiple PersistentVolumeClaims for snapshotting. A key aim is to allow you restore that set of snapshots to new volumes and recover your workload based on a crash consistent recovery point.
This new feature is only supported for CSI volume drivers.
An overview of volume group snapshots
Some storage systems provide the ability to create a crash consistent snapshot of multiple volumes. A group snapshot represents copies made from multiple volumes, that are taken at the same point-in-time. A group snapshot can be used either to rehydrate new volumes (pre-populated with the snapshot data) or to restore existing volumes to a previous state (represented by the snapshots).
Why add volume group snapshots to Kubernetes?
The Kubernetes volume plugin system already provides a powerful abstraction that automates the provisioning, attaching, mounting, resizing, and snapshotting of block and file storage.
Underpinning all these features is the Kubernetes goal of workload portability: Kubernetes aims to create an abstraction layer between distributed applications and underlying clusters so that applications can be agnostic to the specifics of the cluster they run on and application deployment requires no cluster specific knowledge.
There was already a VolumeSnapshot API that provides the ability to take a snapshot of a persistent volume to protect against data loss or data corruption. However, there are other snapshotting functionalities not covered by the VolumeSnapshot API.
Some storage systems support consistent group snapshots that allow a snapshot to be taken from multiple volumes at the same point-in-time to achieve write order consistency. This can be useful for applications that contain multiple volumes. For example, an application may have data stored in one volume and logs stored in another volume. If snapshots for the data volume and the logs volume are taken at different times, the application will not be consistent and will not function properly if it is restored from those snapshots when a disaster strikes.
It is true that you can quiesce the application first, take an individual snapshot from each volume that is part of the application one after the other, and then unquiesce the application after all the individual snapshots are taken. This way, you would get application consistent snapshots.
However, sometimes the application quiesce can be so time consuming that you want to do it less frequently, or it may not be possible to quiesce an application at all. For example, a user may want to run weekly backups with application quiesce and nightly backups without application quiesce but with consistent group support which provides crash consistency across all volumes in the group.
Kubernetes APIs for volume group snapshots
Kubernetes' support for volume group snapshots relies on three API kinds that are used for managing snapshots:
- VolumeGroupSnapshot
- Created by a Kubernetes user (or perhaps by your own automation) to request creation of a volume group snapshot for multiple persistent volume claims. It contains information about the volume group snapshot operation such as the timestamp when the volume group snapshot was taken and whether it is ready to use. The creation and deletion of this object represents a desire to create or delete a cluster resource (a group snapshot).
- VolumeGroupSnapshotContent
- Created by the snapshot controller for a dynamically created VolumeGroupSnapshot. It contains information about the volume group snapshot including the volume group snapshot ID. This object represents a provisioned resource on the cluster (a group snapshot). The VolumeGroupSnapshotContent object binds to the VolumeGroupSnapshot for which it was created with a one-to-one mapping.
- VolumeGroupSnapshotClass
- Created by cluster administrators to describe how volume group snapshots should be created, including the driver information, the deletion policy, etc.
These three API kinds are defined as CustomResourceDefinitions (CRDs). These CRDs must be installed in a Kubernetes cluster for a CSI Driver to support volume group snapshots.
What components are needed to support volume group snapshots
Volume group snapshots are implemented in the external-snapshotter repository. Implementing volume group snapshots meant adding or changing several components:
- Added new CustomResourceDefinitions for VolumeGroupSnapshot and two supporting APIs.
- Volume group snapshot controller logic is added to the common snapshot controller.
- Adding logic to make CSI calls into the snapshotter sidecar controller.
The volume snapshot controller and CRDs are deployed once per cluster, while the sidecar is bundled with each CSI driver.
Therefore, it makes sense to deploy the volume snapshot controller and CRDs as a cluster addon.
The Kubernetes project recommends that Kubernetes distributors bundle and deploy the volume snapshot controller and CRDs as part of their Kubernetes cluster management process (independent of any CSI Driver).
What's new in Beta?
-
The VolumeGroupSnapshot feature in CSI spec moved to GA in the v1.11.0 release.
-
The snapshot validation webhook was deprecated in external-snapshotter v8.0.0 and it is now removed. Most of the validation webhook logic was added as validation rules into the CRDs. Minimum required Kubernetes version is 1.25 for these validation rules. One thing in the validation webhook not moved to CRDs is the prevention of creating multiple default volume snapshot classes and multiple default volume group snapshot classes for the same CSI driver. With the removal of the validation webhook, an error will still be raised when dynamically provisioning a VolumeSnapshot or VolumeGroupSnapshot when multiple default volume snapshot classes or multiple default volume group snapshot classes for the same CSI driver exist.
-
The
enable-volumegroup-snapshot
flag in the snapshot-controller and the CSI snapshotter sidecar has been replaced by a feature gate. Since VolumeGroupSnapshot is a new API, the feature moves to Beta but the feature gate is disabled by default. To use this feature, enable the feature gate by adding the flag--feature-gates=CSIVolumeGroupSnapshot=true
when starting the snapshot-controller and the CSI snapshotter sidecar. -
The logic to dynamically create the VolumeGroupSnapshot and its corresponding individual VolumeSnapshot and VolumeSnapshotContent objects are moved from the CSI snapshotter to the common snapshot-controller. New RBAC rules are added to the common snapshot-controller and some RBAC rules are removed from the CSI snapshotter sidecar accordingly.
How do I use Kubernetes volume group snapshots
Creating a new group snapshot with Kubernetes
Once a VolumeGroupSnapshotClass object is defined and you have volumes you want to snapshot together, you may request a new group snapshot by creating a VolumeGroupSnapshot object.
The source of the group snapshot specifies whether the underlying group snapshot should be dynamically created or if a pre-existing VolumeGroupSnapshotContent should be used.
A pre-existing VolumeGroupSnapshotContent is created by a cluster administrator. It contains the details of the real volume group snapshot on the storage system which is available for use by cluster users.
One of the following members in the source of the group snapshot must be set.
selector
- a label query over PersistentVolumeClaims that are to be grouped together for snapshotting. This selector will be used to match the label added to a PVC.volumeGroupSnapshotContentName
- specifies the name of a pre-existing VolumeGroupSnapshotContent object representing an existing volume group snapshot.
Dynamically provision a group snapshot
In the following example, there are two PVCs.
NAME STATUS VOLUME CAPACITY ACCESS MODES STORAGECLASS VOLUMEATTRIBUTESCLASS AGE
pvc-0 Bound pvc-6e1f7d34-a5c5-4548-b104-01e72c72b9f2 100Mi RWO csi-hostpath-sc <unset> 2m15s
pvc-1 Bound pvc-abc640b3-2cc1-4c56-ad0c-4f0f0e636efa 100Mi RWO csi-hostpath-sc <unset> 2m7s
Label the PVCs.
% kubectl label pvc pvc-0 group=myGroup
persistentvolumeclaim/pvc-0 labeled
% kubectl label pvc pvc-1 group=myGroup
persistentvolumeclaim/pvc-1 labeled
For dynamic provisioning, a selector must be set so that the snapshot controller can find PVCs with the matching labels to be snapshotted together.
apiVersion: groupsnapshot.storage.k8s.io/v1beta1
kind: VolumeGroupSnapshot
metadata:
name: snapshot-daily-20241217
namespace: demo-namespace
spec:
volumeGroupSnapshotClassName: csi-groupSnapclass
source:
selector:
matchLabels:
group: myGroup
In the VolumeGroupSnapshot spec, a user can specify the VolumeGroupSnapshotClass which has the information about which CSI driver should be used for creating the group snapshot. A VolumGroupSnapshotClass is required for dynamic provisioning.
apiVersion: groupsnapshot.storage.k8s.io/v1beta1
kind: VolumeGroupSnapshotClass
metadata:
name: csi-groupSnapclass
annotations:
kubernetes.io/description: "Example group snapshot class"
driver: example.csi.k8s.io
deletionPolicy: Delete
As a result of the volume group snapshot creation, a corresponding VolumeGroupSnapshotContent object will be created with a volumeGroupSnapshotHandle pointing to a resource on the storage system.
Two individual volume snapshots will be created as part of the volume group snapshot creation.
NAME READYTOUSE SOURCEPVC RESTORESIZE SNAPSHOTCONTENT AGE
snapshot-0962a745b2bf930bb385b7b50c9b08af471f1a16780726de19429dd9c94eaca0 true pvc-0 100Mi snapcontent-0962a745b2bf930bb385b7b50c9b08af471f1a16780726de19429dd9c94eaca0 16m
snapshot-da577d76bd2106c410616b346b2e72440f6ec7b12a75156263b989192b78caff true pvc-1 100Mi snapcontent-da577d76bd2106c410616b346b2e72440f6ec7b12a75156263b989192b78caff 16m
Importing an existing group snapshot with Kubernetes
To import a pre-existing volume group snapshot into Kubernetes, you must also import the corresponding individual volume snapshots.
Identify the individual volume snapshot handles, manually construct a VolumeSnapshotContent object first, then create a VolumeSnapshot object pointing to the VolumeSnapshotContent object. Repeat this for every individual volume snapshot.
Then manually create a VolumeGroupSnapshotContent object, specifying the volumeGroupSnapshotHandle and individual volumeSnapshotHandles already existing on the storage system.
apiVersion: groupsnapshot.storage.k8s.io/v1beta1
kind: VolumeGroupSnapshotContent
metadata:
name: static-group-content
spec:
deletionPolicy: Delete
driver: hostpath.csi.k8s.io
source:
groupSnapshotHandles:
volumeGroupSnapshotHandle: e8779136-a93e-11ef-9549-66940726f2fd
volumeSnapshotHandles:
- e8779147-a93e-11ef-9549-66940726f2fd
- e8783cd0-a93e-11ef-9549-66940726f2fd
volumeGroupSnapshotRef:
name: static-group-snapshot
namespace: demo-namespace
After that create a VolumeGroupSnapshot object pointing to the VolumeGroupSnapshotContent object.
apiVersion: groupsnapshot.storage.k8s.io/v1beta1
kind: VolumeGroupSnapshot
metadata:
name: static-group-snapshot
namespace: demo-namespace
spec:
source:
volumeGroupSnapshotContentName: static-group-content
How to use group snapshot for restore in Kubernetes
At restore time, the user can request a new PersistentVolumeClaim to be created from a VolumeSnapshot object that is part of a VolumeGroupSnapshot. This will trigger provisioning of a new volume that is pre-populated with data from the specified snapshot. The user should repeat this until all volumes are created from all the snapshots that are part of a group snapshot.
apiVersion: v1
kind: PersistentVolumeClaim
metadata:
name: examplepvc-restored-2024-12-17
namespace: demo-namespace
spec:
storageClassName: example-foo-nearline
dataSource:
name: snapshot-0962a745b2bf930bb385b7b50c9b08af471f1a16780726de19429dd9c94eaca0
kind: VolumeSnapshot
apiGroup: snapshot.storage.k8s.io
accessModes:
- ReadWriteOncePod
resources:
requests:
storage: 100Mi # must be enough storage to fit the existing snapshot
As a storage vendor, how do I add support for group snapshots to my CSI driver?
To implement the volume group snapshot feature, a CSI driver must:
- Implement a new group controller service.
- Implement group controller RPCs:
CreateVolumeGroupSnapshot
,DeleteVolumeGroupSnapshot
, andGetVolumeGroupSnapshot
. - Add group controller capability
CREATE_DELETE_GET_VOLUME_GROUP_SNAPSHOT
.
See the CSI spec and the Kubernetes-CSI Driver Developer Guide for more details.
As mentioned earlier, it is strongly recommended that Kubernetes distributors bundle and deploy the volume snapshot controller and CRDs as part of their Kubernetes cluster management process (independent of any CSI Driver).
As part of this recommended deployment process, the Kubernetes team provides a number of sidecar (helper) containers, including the external-snapshotter sidecar container which has been updated to support volume group snapshot.
The external-snapshotter watches the Kubernetes API server for VolumeGroupSnapshotContent objects, and triggers CreateVolumeGroupSnapshot
and DeleteVolumeGroupSnapshot
operations against a CSI endpoint.
What are the limitations?
The beta implementation of volume group snapshots for Kubernetes has the following limitations:
- Does not support reverting an existing PVC to an earlier state represented by a snapshot (only supports provisioning a new volume from a snapshot).
- No application consistency guarantees beyond any guarantees provided by the storage system (e.g. crash consistency). See this doc for more discussions on application consistency.
What's next?
Depending on feedback and adoption, the Kubernetes project plans to push the volume group snapshot implementation to general availability (GA) in a future release.
How can I learn more?
- The design spec for the volume group snapshot feature.
- The code repository for volume group snapshot APIs and controller.
- CSI documentation on the group snapshot feature.
How do I get involved?
This project, like all of Kubernetes, is the result of hard work by many contributors from diverse backgrounds working together. On behalf of SIG Storage, I would like to offer a huge thank you to the contributors who stepped up these last few quarters to help the project reach beta:
- Ben Swartzlander (bswartz)
- Cici Huang (cici37)
- Hemant Kumar (gnufied)
- James Defelice (jdef)
- Jan Šafránek (jsafrane)
- Madhu Rajanna (Madhu-1)
- Manish M Yathnalli (manishym)
- Michelle Au (msau42)
- Niels de Vos (nixpanic)
- Leonardo Cecchi (leonardoce)
- Rakshith R (Rakshith-R)
- Raunak Shah (RaunakShah)
- Saad Ali (saad-ali)
- Xing Yang (xing-yang)
- Yati Padia (yati1998)
For those interested in getting involved with the design and development of CSI or any part of the Kubernetes Storage system, join the Kubernetes Storage Special Interest Group (SIG). We always welcome new contributors.
We also hold regular Data Protection Working Group meetings. New attendees are welcome to join our discussions.
18 Dec 2024 12:00am GMT
17 Dec 2024
Kubernetes Blog
Enhancing Kubernetes API Server Efficiency with API Streaming
Managing Kubernetes clusters efficiently is critical, especially as their size is growing. A significant challenge with large clusters is the memory overhead caused by list requests.
In the existing implementation, the kube-apiserver processes list requests by assembling the entire response in-memory before transmitting any data to the client. But what if the response body is substantial, say hundreds of megabytes? Additionally, imagine a scenario where multiple list requests flood in simultaneously, perhaps after a brief network outage. While API Priority and Fairness has proven to reasonably protect kube-apiserver from CPU overload, its impact is visibly smaller for memory protection. This can be explained by the differing nature of resource consumption by a single API request - the CPU usage at any given time is capped by a constant, whereas memory, being uncompressible, can grow proportionally with the number of processed objects and is unbounded. This situation poses a genuine risk, potentially overwhelming and crashing any kube-apiserver within seconds due to out-of-memory (OOM) conditions. To better visualize the issue, let's consider the below graph.
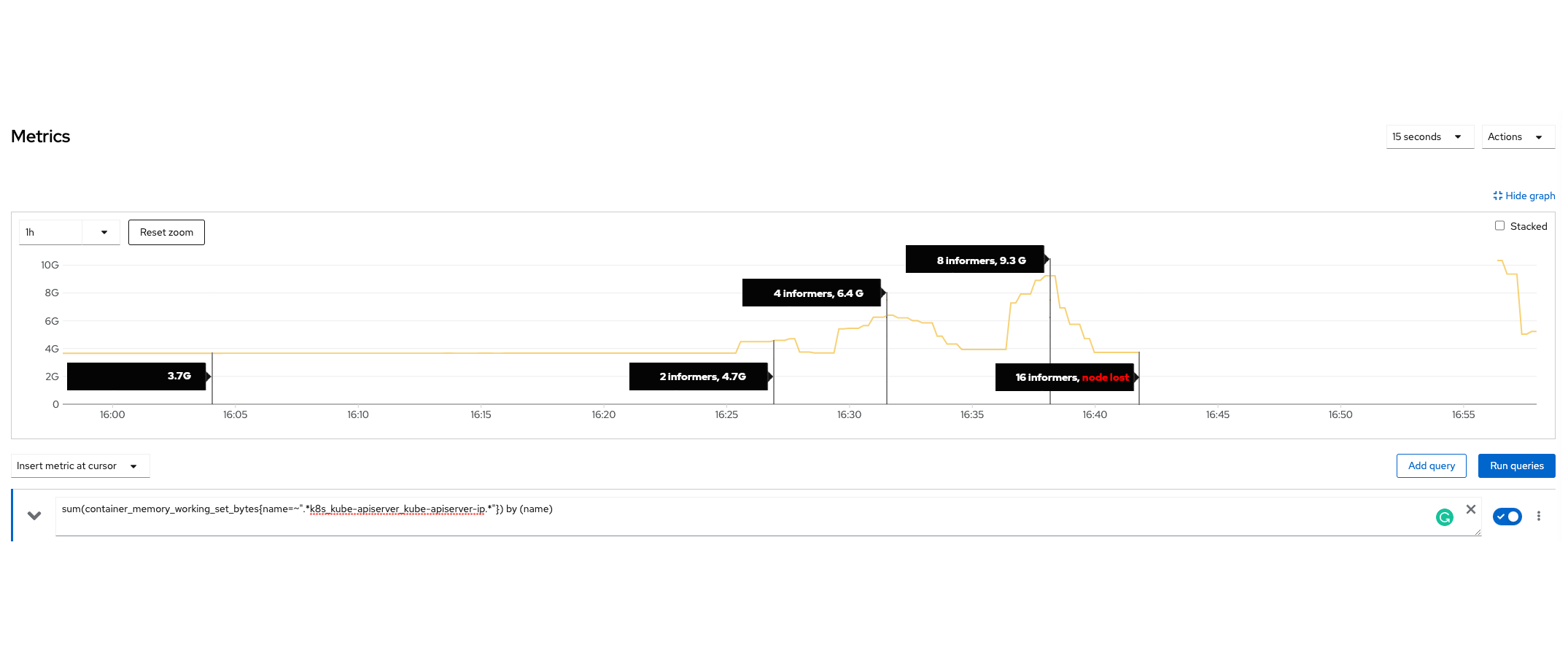
The graph shows the memory usage of a kube-apiserver during a synthetic test. (see the synthetic test section for more details). The results clearly show that increasing the number of informers significantly boosts the server's memory consumption. Notably, at approximately 16:40, the server crashed when serving only 16 informers.
Why does kube-apiserver allocate so much memory for list requests?
Our investigation revealed that this substantial memory allocation occurs because the server before sending the first byte to the client must:
- fetch data from the database,
- deserialize the data from its stored format,
- and finally construct the final response by converting and serializing the data into a client requested format
This sequence results in significant temporary memory consumption. The actual usage depends on many factors like the page size, applied filters (e.g. label selectors), query parameters, and sizes of individual objects.
Unfortunately, neither API Priority and Fairness nor Golang's garbage collection or Golang memory limits can prevent the system from exhausting memory under these conditions. The memory is allocated suddenly and rapidly, and just a few requests can quickly deplete the available memory, leading to resource exhaustion.
Depending on how the API server is run on the node, it might either be killed through OOM by the kernel when exceeding the configured memory limits during these uncontrolled spikes, or if limits are not configured it might have even worse impact on the control plane node. And worst, after the first API server failure, the same requests will likely hit another control plane node in an HA setup with probably the same impact. Potentially a situation that is hard to diagnose and hard to recover from.
Streaming list requests
Today, we're excited to announce a major improvement. With the graduation of the watch list feature to beta in Kubernetes 1.32, client-go users can opt-in (after explicitly enabling WatchListClient
feature gate) to streaming lists by switching from list to (a special kind of) watch requests.
Watch requests are served from the watch cache, an in-memory cache designed to improve scalability of read operations. By streaming each item individually instead of returning the entire collection, the new method maintains constant memory overhead. The API server is bound by the maximum allowed size of an object in etcd plus a few additional allocations. This approach drastically reduces the temporary memory usage compared to traditional list requests, ensuring a more efficient and stable system, especially in clusters with a large number of objects of a given type or large average object sizes where despite paging memory consumption used to be high.
Building on the insight gained from the synthetic test (see the synthetic test, we developed an automated performance test to systematically evaluate the impact of the watch list feature. This test replicates the same scenario, generating a large number of Secrets with a large payload, and scaling the number of informers to simulate heavy list request patterns. The automated test is executed periodically to monitor memory usage of the server with the feature enabled and disabled.
The results showed significant improvements with the watch list feature enabled. With the feature turned on, the kube-apiserver's memory consumption stabilized at approximately 2 GB. By contrast, with the feature disabled, memory usage increased to approximately 20GB, a 10x increase! These results confirm the effectiveness of the new streaming API, which reduces the temporary memory footprint.
Enabling API Streaming for your component
Upgrade to Kubernetes 1.32. Make sure your cluster uses etcd in version 3.4.31+ or 3.5.13+. Change your client software to use watch lists. If your client code is written in Golang, you'll want to enable WatchListClient
for client-go. For details on enabling that feature, read Introducing Feature Gates to Client-Go: Enhancing Flexibility and Control.
What's next?
In Kubernetes 1.32, the feature is enabled in kube-controller-manager by default despite its beta state. This will eventually be expanded to other core components like kube-scheduler or kubelet; once the feature becomes generally available, if not earlier. Other 3rd-party components are encouraged to opt-in to the feature during the beta phase, especially when they are at risk of accessing a large number of resources or kinds with potentially large object sizes.
For the time being, API Priority and Fairness assigns a reasonable small cost to list requests. This is necessary to allow enough parallelism for the average case where list requests are cheap enough. But it does not match the spiky exceptional situation of many and large objects. Once the majority of the Kubernetes ecosystem has switched to watch list, the list cost estimation can be changed to larger values without risking degraded performance in the average case, and with that increasing the protection against this kind of requests that can still hit the API server in the future.
The synthetic test
In order to reproduce the issue, we conducted a manual test to understand the impact of list requests on kube-apiserver memory usage. In the test, we created 400 Secrets, each containing 1 MB of data, and used informers to retrieve all Secrets.
The results were alarming, only 16 informers were needed to cause the test server to run out of memory and crash, demonstrating how quickly memory consumption can grow under such conditions.
Special shout out to @deads2k for his help in shaping this feature.
17 Dec 2024 12:00am GMT
16 Dec 2024
Kubernetes Blog
Kubernetes v1.32 Adds A New CPU Manager Static Policy Option For Strict CPU Reservation
In Kubernetes v1.32, after years of community discussion, we are excited to introduce a strict-cpu-reservation
option for the CPU Manager static policy. This feature is currently in alpha, with the associated policy hidden by default. You can only use the policy if you explicitly enable the alpha behavior in your cluster.
Understanding the feature
The CPU Manager static policy is used to reduce latency or improve performance. The reservedSystemCPUs
defines an explicit CPU set for OS system daemons and kubernetes system daemons. This option is designed for Telco/NFV type use cases where uncontrolled interrupts/timers may impact the workload performance. you can use this option to define the explicit cpuset for the system/kubernetes daemons as well as the interrupts/timers, so the rest CPUs on the system can be used exclusively for workloads, with less impact from uncontrolled interrupts/timers. More details of this parameter can be found on the Explicitly Reserved CPU List page.
If you want to protect your system daemons and interrupt processing, the obvious way is to use the reservedSystemCPUs
option.
However, until the Kubernetes v1.32 release, this isolation was only implemented for guaranteed pods that made requests for a whole number of CPUs. At pod admission time, the kubelet only compares the CPU requests against the allocatable CPUs. In Kubernetes, limits can be higher than the requests; the previous implementation allowed burstable and best-effort pods to use up the capacity of reservedSystemCPUs
, which could then starve host OS services of CPU - and we know that people saw this in real life deployments. The existing behavior also made benchmarking (for both infrastructure and workloads) results inaccurate.
When this new strict-cpu-reservation
policy option is enabled, the CPU Manager static policy will not allow any workload to use the reserved system CPU cores.
Enabling the feature
To enable this feature, you need to turn on both the CPUManagerPolicyAlphaOptions
feature gate and the strict-cpu-reservation
policy option. And you need to remove the /var/lib/kubelet/cpu_manager_state
file if it exists and restart kubelet.
With the following kubelet configuration:
kind: KubeletConfiguration
apiVersion: kubelet.config.k8s.io/v1beta1
featureGates:
...
CPUManagerPolicyOptions: true
CPUManagerPolicyAlphaOptions: true
cpuManagerPolicy: static
cpuManagerPolicyOptions:
strict-cpu-reservation: "true"
reservedSystemCPUs: "0,32,1,33,16,48"
...
When strict-cpu-reservation
is not set or set to false:
# cat /var/lib/kubelet/cpu_manager_state
{"policyName":"static","defaultCpuSet":"0-63","checksum":1058907510}
When strict-cpu-reservation
is set to true:
# cat /var/lib/kubelet/cpu_manager_state
{"policyName":"static","defaultCpuSet":"2-15,17-31,34-47,49-63","checksum":4141502832}
Monitoring the feature
You can monitor the feature impact by checking the following CPU Manager counters:
cpu_manager_shared_pool_size_millicores
: report shared pool size, in millicores (e.g. 13500m)cpu_manager_exclusive_cpu_allocation_count
: report exclusively allocated cores, counting full cores (e.g. 16)
Your best-effort workloads may starve if the cpu_manager_shared_pool_size_millicores
count is zero for prolonged time.
We believe any pod that is required for operational purpose like a log forwarder should not run as best-effort, but you can review and adjust the amount of CPU cores reserved as needed.
Conclusion
Strict CPU reservation is critical for Telco/NFV use cases. It is also a prerequisite for enabling the all-in-one type of deployments where workloads are placed on nodes serving combined control+worker+storage roles.
We want you to start using the feature and looking forward to your feedback.
Further reading
Please check out the Control CPU Management Policies on the Node task page to learn more about the CPU Manager, and how it fits in relation to the other node-level resource managers.
Getting involved
This feature is driven by the SIG Node. If you are interested in helping develop this feature, sharing feedback, or participating in any other ongoing SIG Node projects, please attend the SIG Node meeting for more details.
16 Dec 2024 12:00am GMT
13 Dec 2024
Kubernetes Blog
Kubernetes v1.32: Memory Manager Goes GA
With Kubernetes 1.32, the memory manager has officially graduated to General Availability (GA), marking a significant milestone in the journey toward efficient and predictable memory allocation for containerized applications. Since Kubernetes v1.22, where it graduated to beta, the memory manager has proved itself reliable, stable and a good complementary feature for the CPU Manager.
As part of kubelet's workload admission process, the memory manager provides topology hints to optimize memory allocation and alignment. This enables users to allocate exclusive memory for Pods in the Guaranteed QoS class. More details about the process can be found in the memory manager goes to beta blog.
Most of the changes introduced since the Beta are bug fixes, internal refactoring and observability improvements, such as metrics and better logging.
Observability improvements
As part of the effort to increase the observability of memory manager, new metrics have been added to provide some statistics on memory allocation patterns.
-
memory_manager_pinning_requests_total - tracks the number of times the pod spec required the memory manager to pin memory pages.
-
memory_manager_pinning_errors_total - tracks the number of times the pod spec required the memory manager to pin memory pages, but the allocation failed.
Improving memory manager reliability and consistency
The kubelet does not guarantee pod ordering when admitting pods after a restart or reboot.
In certain edge cases, this behavior could cause the memory manager to reject some pods, and in more extreme cases, it may cause kubelet to fail upon restart.
Previously, the beta implementation lacked certain checks and logic to prevent these issues.
To stabilize the memory manager for general availability (GA) readiness, small but critical refinements have been made to the algorithm, improving its robustness and handling of edge cases.
Future development
There is more to come for the future of Topology Manager in general, and memory manager in particular. Notably, ongoing efforts are underway to extend memory manager support to Windows, enabling CPU and memory affinity on a Windows operating system.
Getting involved
This feature is driven by the SIG Node community. Please join us to connect with the community and share your ideas and feedback around the above feature and beyond. We look forward to hearing from you!
13 Dec 2024 12:00am GMT
12 Dec 2024
Kubernetes Blog
Kubernetes v1.32: QueueingHint Brings a New Possibility to Optimize Pod Scheduling
The Kubernetes scheduler is the core component that selects the nodes on which new Pods run. The scheduler processes these new Pods one by one. Therefore, the larger your clusters, the more important the throughput of the scheduler becomes.
Over the years, Kubernetes SIG Scheduling has improved the throughput of the scheduler in multiple enhancements. This blog post describes a major improvement to the scheduler in Kubernetes v1.32: a scheduling context element named QueueingHint. This page provides background knowledge of the scheduler and explains how QueueingHint improves scheduling throughput.
Scheduling queue
The scheduler stores all unscheduled Pods in an internal component called the scheduling queue.
The scheduling queue consists of the following data structures:
- ActiveQ: holds newly created Pods or Pods that are ready to be retried for scheduling.
- BackoffQ: holds Pods that are ready to be retried but are waiting for a backoff period to end. The backoff period depends on the number of unsuccessful scheduling attempts performed by the scheduler on that Pod.
- Unschedulable Pod Pool: holds Pods that the scheduler won't attempt to schedule for one of the following reasons:
- The scheduler previously attempted and was unable to schedule the Pods. Since that attempt, the cluster hasn't changed in a way that could make those Pods schedulable.
- The Pods are blocked from entering the scheduling cycles by PreEnqueue Plugins, for example, they have a scheduling gate, and get blocked by the scheduling gate plugin.
Scheduling framework and plugins
The Kubernetes scheduler is implemented following the Kubernetes scheduling framework.
And, all scheduling features are implemented as plugins (e.g., Pod affinity is implemented in the InterPodAffinity
plugin.)
The scheduler processes pending Pods in phases called cycles as follows:
-
Scheduling cycle: the scheduler takes pending Pods from the activeQ component of the scheduling queue one by one. For each Pod, the scheduler runs the filtering/scoring logic from every scheduling plugin. The scheduler then decides on the best node for the Pod, or decides that the Pod can't be scheduled at that time.
If the scheduler decides that a Pod can't be scheduled, that Pod enters the Unschedulable Pod Pool component of the scheduling queue. However, if the scheduler decides to place the Pod on a node, the Pod goes to the binding cycle.
-
Binding cycle: the scheduler communicates the node placement decision to the Kubernetes API server. This operation bounds the Pod to the selected node.
Aside from some exceptions, most unscheduled Pods enter the unschedulable pod pool after each scheduling cycle. The Unschedulable Pod Pool component is crucial because of how the scheduling cycle processes Pods one by one. If the scheduler had to constantly retry placing unschedulable Pods, instead of offloading those Pods to the Unschedulable Pod Pool, multiple scheduling cycles would be wasted on those Pods.
Improvements to retrying Pod scheduling with QueuingHint
Unschedulable Pods only move back into the ActiveQ or BackoffQ components of the scheduling queue if changes in the cluster might allow the scheduler to place those Pods on nodes.
Prior to v1.32, each plugin registered which cluster changes could solve their failures, an object creation, update, or deletion in the cluster (called cluster events), with EnqueueExtensions
(EventsToRegister
), and the scheduling queue retries a pod with an event that is registered by a plugin that rejected the pod in a previous scheduling cycle.
Additionally, we had an internal feature called preCheck
, which helped further filtering of events for efficiency, based on Kubernetes core scheduling constraints; For example, preCheck
could filter out node-related events when the node status is NotReady
.
However, we had two issues for those approaches:
- Requeueing with events was too broad, could lead to scheduling retries for no reason.
- A new scheduled Pod might solve the
InterPodAffinity
's failure, but not all of them do. For example, if a new Pod is created, but without a label matchingInterPodAffinity
of the unschedulable pod, the pod wouldn't be schedulable.
- A new scheduled Pod might solve the
preCheck
relied on the logic of in-tree plugins and was not extensible to custom plugins, like in issue #110175.
Here QueueingHints come into play; a QueueingHint subscribes to a particular kind of cluster event, and make a decision about whether each incoming event could make the Pod schedulable.
For example, consider a Pod named pod-a
that has a required Pod affinity. pod-a
was rejected in the scheduling cycle by the InterPodAffinity
plugin because no node had an existing Pod that matched the Pod affinity specification for pod-a
.
A diagram showing the scheduling queue and pod-a rejected by InterPodAffinity plugin
pod-a
moves into the Unschedulable Pod Pool. The scheduling queue records which plugin caused the scheduling failure for the Pod. For pod-a
, the scheduling queue records that the InterPodAffinity
plugin rejected the Pod.
pod-a
will never be schedulable until the InterPodAffinity failure is resolved. There're some scenarios that the failure could be resolved, one example is an existing running pod gets a label update and becomes matching a Pod affinity. For this scenario, the InterPodAffinity
plugin's QueuingHint
callback function checks every Pod label update that occurs in the cluster. Then, if a Pod gets a label update that matches the Pod affinity requirement of pod-a
, the InterPodAffinity
, plugin's QueuingHint
prompts the scheduling queue to move pod-a
back into the ActiveQ or the BackoffQ component.
A diagram showing the scheduling queue and pod-a being moved by InterPodAffinity QueueingHint
QueueingHint's history and what's new in v1.32
At SIG Scheduling, we have been working on the development of QueueingHint since Kubernetes v1.28.
While QueuingHint isn't user-facing, we implemented the SchedulerQueueingHints
feature gate as a safety measure when we originally added this feature. In v1.28, we implemented QueueingHints with a few in-tree plugins experimentally, and made the feature gate enabled by default.
However, users reported a memory leak, and consequently we disabled the feature gate in a patch release of v1.28. From v1.28 until v1.31, we kept working on the QueueingHint implementation within the rest of the in-tree plugins and fixing bugs.
In v1.32, we made this feature enabled by default again. We finished implementing QueueingHints in all plugins and also identified the cause of the memory leak!
We thank all the contributors who participated in the development of this feature and those who reported and investigated the earlier issues.
Getting involved
These features are managed by Kubernetes SIG Scheduling.
Please join us and share your feedback.
How can I learn more?
12 Dec 2024 12:00am GMT
11 Dec 2024
Kubernetes Blog
Kubernetes v1.32: Penelope
Editors: Matteo Bianchi, Edith Puclla, William Rizzo, Ryota Sawada, Rashan Smith
Announcing the release of Kubernetes v1.32: Penelope!
In line with previous releases, the release of Kubernetes v1.32 introduces new stable, beta, and alpha features. The consistent delivery of high-quality releases underscores the strength of our development cycle and the vibrant support from our community. This release consists of 44 enhancements in total. Of those enhancements, 13 have graduated to Stable, 12 are entering Beta, and 19 have entered in Alpha.
Release theme and logo
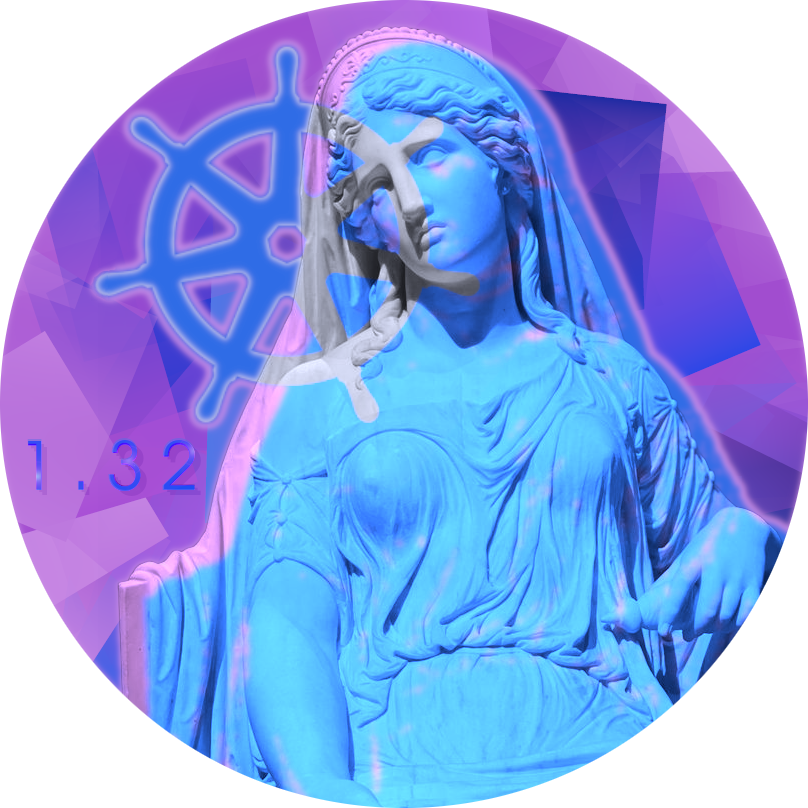
The Kubernetes v1.32 Release Theme is "Penelope".
If Kubernetes is Ancient Greek for "pilot", in this release we start from that origin and reflect on the last 10 years of Kubernetes and our accomplishments: each release cycle is a journey, and just like Penelope, in "The Odyssey",
weaved for 10 years -- each night removing parts of what she had done during the day -- so does each release add new features and removes others, albeit here with a much clearer purpose of constantly improving Kubernetes. With v1.32 being the last release in the year Kubernetes marks its first decade anniversary, we wanted to honour all of those that have been part of the global Kubernetes crew that roams the cloud-native seas through perils and challanges: may we continue to weave the future of Kubernetes together.
Updates to recent key features
A note on DRA enhancements
In this release, like the previous one, the Kubernetes project continues proposing a number of enhancements to the Dynamic Resource Allocation (DRA), a key component of the Kubernetes resource management system. These enhancements aim to improve the flexibility and efficiency of resource allocation for workloads that require specialized hardware, such as GPUs, FPGAs and network adapters. These features are particularly useful for use-cases such as machine learning or high-performance computing applications. The core part enabling DRA Structured parameter support got promoted to beta.
Quality of life improvements on nodes and sidecar containers update
SIG Node has the following highlights that go beyond KEPs:
-
The systemd watchdog capability is now used to restart the kubelet when its health check fails, while also limiting the maximum number of restarts within a given time period. This enhances the reliability of the kubelet. For more details, see pull request #127566.
-
In cases when an image pull back-off error is encountered, the message displayed in the Pod status has been improved to be more human-friendly and to indicate details about why the Pod is in this condition. When an image pull back-off occurs, the error is appended to the
status.containerStatuses[*].state.waiting.message
field in the Pod specification with anImagePullBackOff
value in thereason
field. This change provides you with more context and helps you to identify the root cause of the issue. For more details, see pull request #127918. -
The sidecar containers feature is targeting graduation to Stable in v1.33. To view the remaining work items and feedback from users, see comments in the issue #753.
Highlights of features graduating to Stable
This is a selection of some of the improvements that are now stable following the v1.32 release.
Custom Resource field selectors
Custom resource field selector allows developers to add field selectors to custom resources, mirroring the functionality available for built-in Kubernetes objects. This allows for more efficient and precise filtering of custom resources, promoting better API design practices.
This work was done as a part of KEP #4358, by SIG API Machinery.
Support to size memory backed volumes
This feature makes it possible to dynamically size memory-backed volumes based on Pod resource limits, improving the workload's portability and overall node resource utilization.
This work was done as a part of KEP #1967, by SIG Node.
Bound service account token improvement
The inclusion of the node name in the service account token claims allows users to use such information during authorization and admission (ValidatingAdmissionPolicy). Furthermore this improvement keeps service account credentials from being a privilege escalation path for nodes.
This work was done as part of KEP #4193 by SIG Auth.
Structured authorization configuration
Multiple authorizers can be configured in the API server to allow for structured authorization decisions, with support for CEL match conditions in webhooks. This work was done as part of KEP #3221 by SIG Auth.
Auto remove PVCs created by StatefulSet
PersistentVolumeClaims (PVCs) created by StatefulSets get automatically deleted when no longer needed, while ensuring data persistence during StatefulSet updates and node maintenance. This feature simplifies storage management for StatefulSets and reduces the risk of orphaned PVCs.
This work was done as part of KEP #1847 by SIG Apps.
Highlights of features graduating to Beta
This is a selection of some of the improvements that are now beta following the v1.32 release.
Job API managed-by mechanism
The managedBy
field for Jobs was promoted to beta in the v1.32 release. This feature enables external controllers (like Kueue) to manage Job synchronization, offering greater flexibility and integration with advanced workload management systems.
This work was done as a part of KEP #4368, by SIG Apps.
Only allow anonymous auth for configured endpoints
This feature lets admins specify which endpoints are allowed for anonymous requests. For example, the admin can choose to only allow anonymous access to health endpoints like /healthz
, /livez
, and /readyz
while making sure preventing anonymous access to other cluster endpoints or resources even if a user misconfigures RBAC.
This work was done as a part of KEP #4633, by SIG Auth.
Per-plugin callback functions for accurate requeueing in kube-scheduler enhancements
This feature enhances scheduling throughput with more efficient scheduling retry decisions by per-plugin callback functions (QueueingHint). All plugins now have QueueingHints.
This work was done as a part of KEP #4247, by SIG Scheduling.
Recover from volume expansion failure
This feature lets users recover from volume expansion failure by retrying with a smaller size. This enhancement ensures that volume expansion is more resilient and reliable, reducing the risk of data loss or corruption during the process.
This work was done as a part of KEP #1790, by SIG Storage.
Volume group snapshot
This feature introduces a VolumeGroupSnapshot API, which lets users take a snapshot of multiple volumes together, ensuring data consistency across the volumes.
This work was done as a part of KEP #3476, by SIG Storage.
Structured parameter support
The core part of Dynamic Resource Allocation (DRA), the structured parameter support, got promoted to beta. This allows the kube-scheduler and Cluster Autoscaler to simulate claim allocation directly, without needing a third-party driver. These components can now predict whether resource requests can be fulfilled based on the cluster's current state without actually committing to the allocation. By eliminating the need for a third-party driver to validate or test allocations, this feature improves planning and decision-making for resource distribution, making the scheduling and scaling processes more efficient.
This work was done as a part of KEP #4381, by WG Device Management (a cross functional team containing SIG Node, SIG Scheduling and SIG Autoscaling).
Label and field selector authorization
Label and field selectors can be used in authorization decisions. The node authorizer automatically takes advantage of this to limit nodes to list or watch their pods only. Webhook authorizers can be updated to limit requests based on the label or field selector used.
This work was done as part of KEP #4601 by SIG Auth.
Highlights of new features in Alpha
This is a selection of key improvements introduced as alpha features in the v1.32 release.
Asynchronous preemption in the Kubernetes Scheduler
The Kubernetes scheduler has been enhanced with Asynchronous Preemption, a feature that improves scheduling throughput by handling preemption operations asynchronously. Preemption ensures higher-priority pods get the resources they need by evicting lower-priority ones, but this process previously involved heavy operations like API calls to delete pods, slowing down the scheduler. With this enhancement, such tasks are now processed in parallel, allowing the scheduler to continue scheduling other pods without delays. This improvement is particularly beneficial in clusters with high Pod churn or frequent scheduling failures, ensuring a more efficient and resilient scheduling process.
This work was done as a part of KEP #4832 by SIG Scheduling.
Mutating admission policies using CEL expressions
This feature leverages CEL's object instantiation and JSON Patch strategies, combined with Server Side Apply's merge algorithms. It simplifies policy definition, reduces mutation conflicts, and enhances admission control performance while laying a foundation for more robust, extensible policy frameworks in Kubernetes.
The Kubernetes API server now supports Common Expression Language (CEL)-based Mutating Admission Policies, providing a lightweight, efficient alternative to mutating admission webhooks. With this enhancement, administrators can use CEL to declare mutations like setting labels, defaulting fields, or injecting sidecars with simple, declarative expressions. This approach reduces operational complexity, eliminates the need for webhooks, and integrates directly with the kube-apiserver, offering faster and more reliable in-process mutation handling.
This work was done as a part of KEP #3962 by SIG API Machinery.
Pod-level resource specifications
This enhancement simplifies resource management in Kubernetes by introducing the ability to set resource requests and limits at the Pod level, creating a shared pool that all containers in the Pod can dynamically use. This is particularly valuable for workloads with containers that have fluctuating or bursty resource needs, as it minimizes over-provisioning and improves overall resource efficiency.
By leveraging Linux cgroup settings at the Pod level, Kubernetes ensures that these resource limits are enforced while enabling tightly coupled containers to collaborate more effectively without hitting artificial constraints. Importantly, this feature maintains backward compatibility with existing container-level resource settings, allowing users to adopt it incrementally without disrupting current workflows or existing configurations.
This marks a significant improvement for multi-container pods, as it reduces the operational complexity of managing resource allocations across containers. It also provides a performance boost for tightly integrated applications, such as sidecar architectures, where containers share workloads or depend on each other's availability to perform optimally.
This work was done as part of KEP #2837 by SIG Node.
Allow zero value for sleep action of PreStop hook
This enhancement introduces the ability to set a zero-second sleep duration for the PreStop lifecycle hook in Kubernetes, offering a more flexible and no-op option for resource validation and customization. Previously, attempting to define a zero value for the sleep action resulted in validation errors, restricting its use. With this update, users can configure a zero-second duration as a valid sleep setting, enabling immediate execution and termination behaviors where needed.
The enhancement is backward-compatible, introduced as an opt-in feature controlled by the PodLifecycleSleepActionAllowZero
feature gate. This change is particularly beneficial for scenarios requiring PreStop hooks for validation or admission webhook processing without requiring an actual sleep duration. By aligning with the capabilities of the time.After
Go function, this update simplifies configuration and expands usability for Kubernetes workloads.
This work was done as part of KEP #4818 by SIG Node.
DRA: Standardized network interface data for resource claim status
This enhancement adds a new field that allows drivers to report specific device status data for each allocated object in a ResourceClaim. It also establishes a standardized way to represent networking devices information.
This work was done as a part of KEP #4817, by SIG Network.
New statusz and flagz endpoints for core components
You can enable two new HTTP endpoints, /statusz
and /flagz
, for core components. These enhance cluster debuggability by gaining insight into what versions (e.g. Golang version) that component is running as, along with details about its uptime, and which command line flags that component was executed with; making it easier to diagnose both runtime and configuration issues.
This work was done as part of KEP #4827 and KEP #4828 by SIG Instrumentation.
Windows strikes back!
Support for graceful shutdowns of Windows nodes in Kubernetes clusters has been added. Before this release, Kubernetes provided graceful node shutdown functionality for Linux nodes but lacked equivalent support for Windows. This enhancement enables the kubelet on Windows nodes to handle system shutdown events properly. Doing so, it ensures that Pods running on Windows nodes are gracefully terminated, allowing workloads to be rescheduled without disruption. This improvement enhances the reliability and stability of clusters that include Windows nodes, especially during a planned maintenance or any system updates.
Moreover CPU and memory affinity support has been added for Windows nodes with nodes, with improvements to the CPU manager, memory manager and topology manager.
This work was done respectively as part of KEP #4802 and KEP #4885 by SIG Windows.
Graduations, deprecations, and removals in 1.32
Graduations to Stable
This lists all the features that graduated to stable (also known as general availability). For a full list of updates including new features and graduations from alpha to beta, see the release notes.
This release includes a total of 13 enhancements promoted to Stable:
- Structured Authorization Configuration
- Bound service account token improvements
- Custom Resource Field Selectors
- Retry Generate Name
- Make Kubernetes aware of the LoadBalancer behaviour
- Field
status.hostIPs
added for Pod - Custom profile in kubectl debug
- Memory Manager
- Support to size memory backed volumes
- Improved multi-numa alignment in Topology Manager
- Add job creation timestamp to job annotations
- Add Pod Index Label for StatefulSets and Indexed Jobs
- Auto remove PVCs created by StatefulSet
Deprecations and removals
As Kubernetes develops and matures, features may be deprecated, removed, or replaced with better ones for the project's overall health. See the Kubernetes deprecation and removal policy for more details on this process.
Withdrawal of the old DRA implementation
The enhancement #3063 introduced Dynamic Resource Allocation (DRA) in Kubernetes 1.26.
However, in Kubernetes v1.32, this approach to DRA will be significantly changed. Code related to the original implementation will be removed, leaving KEP #4381 as the "new" base functionality.
The decision to change the existing approach originated from its incompatibility with cluster autoscaling as resource availability was non-transparent, complicating decision-making for both Cluster Autoscaler and controllers. The newly added Structured Parameter model substitutes the functionality.
This removal will allow Kubernetes to handle new hardware requirements and resource claims more predictably, bypassing the complexities of back and forth API calls to the kube-apiserver.
See the enhancement issue #3063 to find out more.
API removals
There is one API removal in Kubernetes v1.32:
- The
flowcontrol.apiserver.k8s.io/v1beta3
API version of FlowSchema and PriorityLevelConfiguration has been removed. To prepare for this, you can edit your existing manifests and rewrite client software to use theflowcontrol.apiserver.k8s.io/v1 API
version, available since v1.29. All existing persisted objects are accessible via the new API. Notable changes in flowcontrol.apiserver.k8s.io/v1beta3 include that the PriorityLevelConfigurationspec.limited.nominalConcurrencyShares
field only defaults to 30 when unspecified, and an explicit value of 0 is not changed to 30.
For more information, refer to the API deprecation guide.
Release notes and upgrade actions required
Check out the full details of the Kubernetes v1.32 release in our release notes.
Availability
Kubernetes v1.32 is available for download on GitHub or on the Kubernetes download page.
To get started with Kubernetes, check out these interactive tutorials or run local Kubernetes clusters using minikube. You can also easily install v1.32 using kubeadm.
Release team
Kubernetes is only possible with the support, commitment, and hard work of its community. Each release team is made up of dedicated community volunteers who work together to build the many pieces that make up the Kubernetes releases you rely on. This requires the specialized skills of people from all corners of our community, from the code itself to its documentation and project management.
We would like to thank the entire release team for the hours spent hard at work to deliver the Kubernetes v1.32 release to our community. The Release Team's membership ranges from first-time shadows to returning team leads with experience forged over several release cycles. A very special thanks goes out our release lead, Frederico Muñoz, for leading the release team so gracefully and handle any matter with the uttermost care, making sure this release was executed smoothly and efficiently. Last but not least a big thanks goes to all the release members - leads and shadows alike - and to the following SIGs for the terrific work and outcome achieved during these 14 weeks of release work:
- SIG Docs - for the fundamental support in docs and blog reviews and continous collaboration with release Comms and Docs;
- SIG k8s Infra and SIG Testing - for the outstanding work in keeping the testing framework in check, along with all the infra components necessary;
- SIG Release and all the release managers - for the incredible support provided throughout the orchestration of the entire release, addressing even the most challenging issues in a graceful and timely manner.
Project velocity
The CNCF K8s DevStats project aggregates a number of interesting data points related to the velocity of Kubernetes and various sub-projects. This includes everything from individual contributions to the number of companies that are contributing and is an illustration of the depth and breadth of effort that goes into evolving this ecosystem.
In the v1.32 release cycle, which ran for 14 weeks (September 9th to December 11th), we saw contributions to Kubernetes from as many as 125 different companies and 559 individuals as of writing.
In the whole Cloud Native ecosystem, the figure goes up to 433 companies counting 2441 total contributors. This sees an increase of 7% more overall contributions compared to the previous release cycle, along with 14% increase in the number of companies involved, showcasing strong interest and community behind the Cloud Native projects.
Source for this data:
By contribution we mean when someone makes a commit, code review, comment, creates an issue or PR, reviews a PR (including blogs and documentation) or comments on issues and PRs.
If you are interested in contributing visit Getting Started on our contributor website.
Check out DevStats to learn more about the overall velocity of the Kubernetes project and community.
Event updates
Explore the upcoming Kubernetes and cloud-native events from March to June 2025, featuring KubeCon and KCD Stay informed and engage with the Kubernetes community.
March 2025
- KCD - Kubernetes Community Days: Beijing, China: In March | Beijing, China
- KCD - Kubernetes Community Days: Guadalajara, Mexico: March 16, 2025 | Guadalajara, Mexico
- KCD - Kubernetes Community Days: Rio de Janeiro, Brazil: March 22, 2025 | Rio de Janeiro, Brazil
April 2025
- KubeCon + CloudNativeCon Europe 2025: April 1-4, 2025 | London, United Kingdom
- KCD - Kubernetes Community Days: Budapest, Hungary: April 23, 2025 | Budapest, Hungary
- KCD - Kubernetes Community Days: Chennai, India: April 26, 2025 | Chennai, India
- KCD - Kubernetes Community Days: Auckland, New Zealand: April 28, 2025 | Auckland, New Zealand
May 2025
- KCD - Kubernetes Community Days: Helsinki, Finland: May 6, 2025 | Helsinki, Finland
- KCD - Kubernetes Community Days: San Francisco, USA: May 8, 2025 | San Francisco, USA
- KCD - Kubernetes Community Days: Austin, USA: May 15, 2025 | Austin, USA
- KCD - Kubernetes Community Days: Seoul, South Korea: May 22, 2025 | Seoul, South Korea
- KCD - Kubernetes Community Days: Istanbul, Turkey: May 23, 2025 | Istanbul, Turkey
- KCD - Kubernetes Community Days: Heredia, Costa Rica: May 31, 2025 | Heredia, Costa Rica
- KCD - Kubernetes Community Days: New York, USA: In May | New York, USA
June 2025
- KCD - Kubernetes Community Days: Bratislava, Slovakia: June 5, 2025 | Bratislava, Slovakia
- KCD - Kubernetes Community Days: Bangalore, India: June 6, 2025 | Bangalore, India
- KubeCon + CloudNativeCon China 2025: June 10-11, 2025 | Hong Kong
- KCD - Kubernetes Community Days: Antigua Guatemala, Guatemala: June 14, 2025 | Antigua Guatemala, Guatemala
- KubeCon + CloudNativeCon Japan 2025: June 16-17, 2025 | Tokyo, Japan
- KCD - Kubernetes Community Days: Nigeria, Africa: June 19, 2025 | Nigeria, Africa
Upcoming release webinar
Join members of the Kubernetes v1.32 release team on Thursday, January 9th 2025 at 5:00 PM (UTC), to learn about the release highlights of this release, as well as deprecations and removals to help plan for upgrades. For more information and registration, visit the event page on the CNCF Online Programs site.
Get involved
The simplest way to get involved with Kubernetes is by joining one of the many Special Interest Groups (SIGs) that align with your interests. Have something you'd like to broadcast to the Kubernetes community? Share your voice at our weekly community meeting, and through the channels below. Thank you for your continued feedback and support.
- Follow us on Bluesky @Kubernetes.io for latest updates
- Join the community discussion on Discuss
- Join the community on Slack
- Post questions (or answer questions) on Stack Overflow
- Share your Kubernetes story
- Read more about what's happening with Kubernetes on the blog
- Learn more about the Kubernetes Release Team
11 Dec 2024 12:00am GMT
21 Nov 2024
Kubernetes Blog
Gateway API v1.2: WebSockets, Timeouts, Retries, and More
Kubernetes SIG Network is delighted to announce the general availability of Gateway API v1.2! This version of the API was released on October 3, and we're delighted to report that we now have a number of conformant implementations of it for you to try out.
Gateway API v1.2 brings a number of new features to the Standard channel (Gateway API's GA release channel), introduces some new experimental features, and inaugurates our new release process - but it also brings two breaking changes that you'll want to be careful of.
Breaking changes
GRPCRoute and ReferenceGrant v1alpha2
removal
Now that the v1
versions of GRPCRoute and ReferenceGrant have graduated to Standard, the old v1alpha2
versions have been removed from both the Standard and Experimental channels, in order to ease the maintenance burden that perpetually supporting the old versions would place on the Gateway API community.
Before upgrading to Gateway API v1.2, you'll want to confirm that any implementations of Gateway API have been upgraded to support the v1 API version of these resources instead of the v1alpha2 API version. Note that even if you've been using v1 in your YAML manifests, a controller may still be using v1alpha2 which would cause it to fail during this upgrade. Additionally, Kubernetes itself goes to some effort to stop you from removing a CRD version that it thinks you're using: check out the release notes for more information about what you need to do to safely upgrade.
Change to .status.supportedFeatures
(experimental)
A much smaller breaking change: .status.supportedFeatures
in a Gateway is now a list of objects instead of a list of strings. The objects have a single name
field, so the translation from the strings is straightforward, but moving to objects permits a lot more flexibility for the future. This stanza is not yet present in the Standard channel.
Graduations to the standard channel
Gateway API 1.2.0 graduates four features to the Standard channel, meaning that they can now be considered generally available. Inclusion in the Standard release channel denotes a high level of confidence in the API surface and provides guarantees of backward compatibility. Of course, as with any other Kubernetes API, Standard channel features can continue to evolve with backward-compatible additions over time, and we certainly expect further refinements and improvements to these new features in the future. For more information on how all of this works, refer to the Gateway API Versioning Policy.
HTTPRoute timeouts
GEP-1742 introduced the timeouts
stanza into HTTPRoute, permitting configuring basic timeouts for HTTP traffic. This is a simple but important feature for proper resilience when handling HTTP traffic, and it is now Standard.
For example, this HTTPRoute configuration sets a timeout of 300ms for traffic to the /face
path:
apiVersion: gateway.networking.k8s.io/v1
kind: HTTPRoute
metadata:
name: face-with-timeouts
namespace: faces
spec:
parentRefs:
- name: my-gateway
kind: Gateway
rules:
- matches:
- path:
type: PathPrefix
value: /face
backendRefs:
- name: face
port: 80
timeouts:
request: 300ms
For more information, check out the HTTP routing documentation. (Note that this applies only to HTTPRoute timeouts. GRPCRoute timeouts are not yet part of Gateway API.)
Gateway infrastructure labels and annotations
Gateway API implementations are responsible for creating the backing infrastructure needed to make each Gateway work. For example, implementations running in a Kubernetes cluster often create Services and Deployments, while cloud-based implementations may be creating cloud load balancer resources. In many cases, it can be helpful to be able to propagate labels or annotations to these generated resources.
In v1.2.0, the Gateway infrastructure
stanza moves to the Standard channel, allowing you to specify labels and annotations for the infrastructure created by the Gateway API controller. For example, if your Gateway infrastructure is running in-cluster, you can specify both Linkerd and Istio injection using the following Gateway configuration, making it simpler for the infrastructure to be incorporated into whichever service mesh you've installed:
apiVersion: gateway.networking.k8s.io/v1
kind: Gateway
metadata:
name: meshed-gateway
namespace: incoming
spec:
gatewayClassName: meshed-gateway-class
listeners:
- name: http-listener
protocol: HTTP
port: 80
infrastructure:
labels:
istio-injection: enabled
annotations:
linkerd.io/inject: enabled
For more information, check out the infrastructure
API reference.
Backend protocol support
Since Kubernetes v1.20, the Service and EndpointSlice resources have supported a stable appProtocol
field to allow users to specify the L7 protocol that Service supports. With the adoption of KEP 3726, Kubernetes now supports three new appProtocol
values:
kubernetes.io/h2c
- HTTP/2 over cleartext as described in RFC7540
kubernetes.io/ws
- WebSocket over cleartext as described in RFC6445
kubernetes.io/wss
- WebSocket over TLS as described in RFC6445
With Gateway API 1.2.0, support for honoring appProtocol
is now Standard. For example, given the following Service:
apiVersion: v1
kind: Service
metadata:
name: websocket-service
namespace: my-namespace
spec:
selector:
app.kubernetes.io/name: websocket-app
ports:
- name: http
port: 80
targetPort: 9376
protocol: TCP
appProtocol: kubernetes.io/ws
then an HTTPRoute that includes this Service as a backendRef
will automatically upgrade the connection to use WebSockets rather than assuming that the connection is pure HTTP.
For more information, check out GEP-1911.
New additions to experimental channel
Named rules for *Route resources
The rules
field in HTTPRoute and GRPCRoute resources can now be named, in order to make it easier to reference the specific rule, for example:
apiVersion: gateway.networking.k8s.io/v1
kind: HTTPRoute
metadata:
name: multi-color-route
namespace: faces
spec:
parentRefs:
- name: my-gateway
kind: Gateway
port: 80
rules:
- name: center-rule
matches:
- path:
type: PathPrefix
value: /color/center
backendRefs:
- name: color-center
port: 80
- name: edge-rule
matches:
- path:
type: PathPrefix
value: /color/edge
backendRefs:
- name: color-edge
port: 80
Logging or status messages can now refer to these two rules as center-rule
or edge-rule
instead of being forced to refer to them by index. For more information, see GEP-995.
HTTPRoute retry support
Gateway API 1.2.0 introduces experimental support for counted HTTPRoute retries. For example, the following HTTPRoute configuration retries requests to the /face
path up to 3 times with a 500ms delay between retries:
apiVersion: gateway.networking.k8s.io/v1
kind: HTTPRoute
metadata:
name: face-with-retries
namespace: faces
spec:
parentRefs:
- name: my-gateway
kind: Gateway
port: 80
rules:
- matches:
- path:
type: PathPrefix
value: /face
backendRefs:
- name: face
port: 80
retry:
codes: [ 500, 502, 503, 504 ]
attempts: 3
backoff: 500ms
For more information, check out GEP 1731.
HTTPRoute percentage-based mirroring
Gateway API has long supported the Request Mirroring feature, which allows sending the same request to multiple backends. In Gateway API 1.2.0, we're introducing percentage-based mirroring, which allows you to specify a percentage of requests to mirror to a different backend. For example, the following HTTPRoute configuration mirrors 42% of requests to the color-mirror
backend:
apiVersion: gateway.networking.k8s.io/v1
kind: HTTPRoute
metadata:
name: color-mirror-route
namespace: faces
spec:
parentRefs:
- name: mirror-gateway
hostnames:
- mirror.example
rules:
- backendRefs:
- name: color
port: 80
filters:
- type: RequestMirror
requestMirror:
backendRef:
name: color-mirror
port: 80
percent: 42 # This value must be an integer.
There's also a fraction
stanza which can be used in place of percent
, to allow for more precise control over exactly what amount of traffic is mirrored, for example:
...
filters:
- type: RequestMirror
requestMirror:
backendRef:
name: color-mirror
port: 80
fraction:
numerator: 1
denominator: 10000
This configuration mirrors 1 in 10,000 requests to the color-mirror
backend, which may be relevant with very high request rates. For more details, see GEP-1731.
Additional backend TLS configuration
This release includes three additions related to TLS configuration for communications between a Gateway and a workload (a backend):
-
A new
backendTLS
field on GatewayThis new field allows you to specify the client certificate that a Gateway should use when connecting to backends.
-
A new
subjectAltNames
field on BackendTLSPolicyPreviously, the
hostname
field was used to configure both the SNI that a Gateway should send to a backend and the identity that should be provided by a certificate. When the newsubjectAltNames
field is specified, any certificate matching at least one of the specified SANs will be considered valid. This is particularly critical for SPIFFE where URI-based SANs may not be valid SNIs. -
A new
options
field on BackendTLSPolicySimilar to the TLS options field on Gateway Listeners, we believe the same concept will be broadly useful for TLS-specific configuration for Backend TLS.
For more information, check out GEP-3135.
More changes
For a full list of the changes included in this release, please refer to the v1.2.0 release notes.
Project updates
Beyond the technical, the v1.2 release also marks a few milestones in the life of the Gateway API project itself.
Release process improvements
Gateway API has never been intended to be a static API, and as more projects use it as a component to build on, it's become clear that we need to bring some more predictability to Gateway API releases. To that end, we're pleased - and a little nervous! - to announce that we've formalized a new release process:
-
Scoping (4-6 weeks): maintainers and community determine the set of features we want to include in the release. A particular emphasis here is getting features out of the Experimental channel - ideally this involves moving them to Standard, but it can also mean removing them.
-
GEP Iteration and Review (5-7 weeks): contributors write or update Gateway Enhancement Proposals (GEPs) for features accepted into the release, with emphasis on getting consensus around the design and graduation criteria of the feature.
-
API Refinement and Documentation (3-5 weeks): contributors implement the features in the Gateway API controllers and write the necessary documentation.
-
SIG Network Review and Release Candidates (2-4 weeks): maintainers get the required upstream review, build release candidates, and release the new version.
Gateway API 1.2.0 was the first release to use the new process, and although there are the usual rough edges of anything new, we believe that it went well. We've already completed the Scoping phase for Gateway API 1.3, with the release expected around the end of January 2025.
gwctl
moves out
The gwctl
CLI tool has moved into its very own repository, https://github.com/kubernetes-sigs/gwctl. gwctl
has proven a valuable tool for the Gateway API community; moving it into its own repository will, we believe, make it easier to maintain and develop. As always, we welcome contributions; while still experimental, gwctl
already helps make working with Gateway API a bit easier - especially for newcomers to the project!
Maintainer changes
Rounding out our changes to the project itself, we're pleased to announce that Mattia Lavacca has joined the ranks of Gateway API Maintainers! We're also sad to announce that Keith Mattix has stepped down as a GAMMA lead - happily, Mike Morris has returned to the role. We're grateful for everything Keith has done, and excited to have Mattia and Mike on board.
Try it out
Unlike other Kubernetes APIs, you don't need to upgrade to the latest version of Kubernetes to get the latest version of Gateway API. As long as you're running Kubernetes 1.26 or later, you'll be able to get up and running with this version of Gateway API.
To try out the API, follow our Getting Started Guide. As of this writing, five implementations are already conformant with Gateway API v1.2. In alphabetical order:
- Cilium v1.17.0-pre.1, Experimental channel
- Envoy Gateway v1.2.0-rc.1, Experimental channel
- Istio v1.24.0-alpha.0, Experimental channel
- Kong v3.2.0-244-gea4944bb0, Experimental channel
- Traefik v3.2, Experimental channel
Get involved
There are lots of opportunities to get involved and help define the future of Kubernetes routing APIs for both ingress and service mesh.
- Check out the user guides to see what use-cases can be addressed.
- Try out one of the existing Gateway controllers.
- Or join us in the community and help us build the future of Gateway API together!
The maintainers would like to thank everyone who's contributed to Gateway API, whether in the form of commits to the repo, discussion, ideas, or general support. We could never have gotten this far without the support of this dedicated and active community.
Related Kubernetes blog articles
- Gateway API v1.1: Service mesh, GRPCRoute, and a whole lot more
- New Experimental Features in Gateway API v1.0 11/2023
- Gateway API v1.0: GA Release 10/2023
- Introducing ingress2gateway; Simplifying Upgrades to Gateway API 10/2023
- Gateway API v0.8.0: Introducing Service Mesh Support 08/2023
21 Nov 2024 5:00pm GMT
How we built a dynamic Kubernetes API Server for the API Aggregation Layer in Cozystack
Hi there! I'm Andrei Kvapil, but you might know me as @kvaps in communities dedicated to Kubernetes and cloud-native tools. In this article, I want to share how we implemented our own extension api-server in the open-source PaaS platform, Cozystack.
Kubernetes truly amazes me with its powerful extensibility features. You're probably already familiar with the controller concept and frameworks like kubebuilder and operator-sdk that help you implement it. In a nutshell, they allow you to extend your Kubernetes cluster by defining custom resources (CRDs) and writing additional controllers that handle your business logic for reconciling and managing these kinds of resources. This approach is well-documented, with a wealth of information available online on how to develop your own operators.
However, this is not the only way to extend the Kubernetes API. For more complex scenarios such as implementing imperative logic, managing subresources, and dynamically generating responses-the Kubernetes API aggregation layer provides an effective alternative. Through the aggregation layer, you can develop a custom extension API server and seamlessly integrate it within the broader Kubernetes API framework.
In this article, I will explore the API aggregation layer, the types of challenges it is well-suited to address, cases where it may be less appropriate, and how we utilized this model to implement our own extension API server in Cozystack.
What Is the API Aggregation Layer?
First, let's get definitions straight to avoid any confusion down the road. The API aggregation layer is a feature in Kubernetes, while an extension api-server is a specific implementation of an API server for the aggregation layer. An extension API server is just like the standard Kubernetes API server, except it runs separately and handles requests for your specific resource types.
So, the aggregation layer lets you write your own extension API server, integrate it easily into Kubernetes, and directly process requests for resources in a certain group. Unlike the CRD mechanism, the extension API is registered in Kubernetes as an APIService, telling Kubernetes to consider this new API server and acknowledge that it serves certain APIs.
You can execute this command to list all registered apiservices:
kubectl get apiservices.apiregistration.k8s.io
Example APIService:
NAME SERVICE AVAILABLE AGE
v1alpha1.apps.cozystack.io cozy-system/cozystack-api True 7h29m
As soon as the Kubernetes api-server receives requests for resources in the group v1alpha1.apps.cozystack.io
, it redirects all those requests to our extension api-server, which can handle them based on the business logic we've built into it.
When to use the API Aggregation Layer
The API Aggregation Layer helps solve several issues where the usual CRD mechanism might not enough. Let's break them down.
Imperative Logic and Subresources
Besides regular resources, Kubernetes also has something called subresources.
In Kubernetes, subresources are additional actions or operations you can perform on primary resources (like Pods, Deployments, Services) via the Kubernetes API. They provide interfaces to manage specific aspects of resources without affecting the entire object.
A simple example is status
, which is traditionally exposed as a separate subresource that you can access independently from the parent object. The status
field isn't meant to be changed
But beyond /status
, Pods in Kubernetes also have subresources like /exec
, /portforward
, and /log
. Interestingly, instead of the usual declarative resources in Kubernetes, these represent endpoints for imperative operations like viewing logs, proxying connections, executing commands in a running container, and so on.
To support such imperative commands on your own API, you need implement an extension API and an extension API server. Here are some well-known examples:
- KubeVirt: An add-on for Kubernetes that extends its API capabilities to run traditional virtual machines. The extension api-server created as part of KubeVirt handles subresources like
/restart
,/console
, and/vnc
for virtual machines. - Knative: A Kubernetes add-on that extends its capabilities for serverless computing, implementing the
/scale
subresource to set up autoscaling for its resource types.
By the way, even though subresource logic in Kubernetes can be imperative, you can manage access to them declaratively using Kubernetes standard RBAC model.
For example this way you can control access to the /log
and /exec
subresources of the Pod kind:
kind: Role
apiVersion: rbac.authorization.k8s.io/v1
metadata:
namespace: default
name: pod-and-pod-logs-reader
rules:
- apiGroups: [""]
resources: ["pods", "pods/log"]
verbs: ["get", "list"]
- apiGroups: [""]
resources: ["pods/exec"]
verbs: ["create"]
You're not tied to use etcd
Usually, the Kubernetes API server uses etcd for its backend. However, implementing your own API server doesn't lock you into using only etcd. If it doesn't make sense to store your server's state in etcd, you can store information in any other system and generate responses on the fly. Here are a few cases to illustrate:
-
metrics-server is a standard extension for Kubernetes which allows you to view real-time metrics of your nodes and pods. It defines alternative Pod and Node kinds in its own metrics.k8s.io API. Requests to these resources are translated into metrics directly from Kubelet. So when you run
kubectl top node
orkubectl top pod
, metrics-server fetches metrics from cAdvisor in real-time. It then returns these metrics to you. Since the information is generated in real-time and is only relevant at the moment of the request, there is no need to store it in etcd. This approach saves resources. -
If needed, you can use a backend other than etcd. You can even implement a Kubernetes-compatible API for it. For example, if you use Postgres, you can create a transparent representation of its entities in the Kubernetes API. Eg. databases, users, and grants within Postgres would appear as regular Kubernetes resources, thanks to your extension API server. You could manage them using
kubectl
or any other Kubernetes-compatible tool. Unlike controllers, which implement business logic using custom resources and reconciliation methods, an extension API server eliminates the need for separate controllers for every kind. This means you don't have to sync state between the Kubernetes API and your backend.
One-Time resources
-
Kubernetes has a special API used to provide users with information about their permissions. This is implemented using the SelfSubjectAccessReview API. One unusual detail of these resources is that you can't view them using get or list verbs. You can only create them (using the create verb) and receive output with information about what you have access to at that moment.
If you try to run
kubectl get selfsubjectaccessreviews
directly, you'll just get an error like this:Error from server (MethodNotAllowed): the server does not allow this method on the requested resource
The reason is that the Kubernetes API server doesn't support any other interaction with this type of resource (you can only CREATE them).
The SelfSubjectAccessReview API supports commands such as:
kubectl auth can-i create deployments --namespace dev
When you run the command above,
kubectl
creates a SelfSubjectAccessReview using the Kubernetes API. This allows Kubernetes to fetch a list of possible permissions for your user. Kubernetes then generates a personalized response to your request in real-time. This logic is different from a scenario where this resource is simply stored in etcd. -
Similarly, in KubeVirt's CDI (Containerized Data Importer) extension, which allows file uploads into a PVC from a local machine using the
virtctl
tool, a special token is required before the upload process begins. This token is generated by creating an UploadTokenRequest resource via the Kubernetes API. Kubernetes routes (proxies) all UploadTokenRequest resource creation requests to the CDI extension API server, which generates and returns the token in response.
Full control over conversion, validation, and output formatting
-
Your own API server can have all the capabilities of the vanilla Kubernetes API server. The resources you create in your API server can be validated immediately on the server side without additional webhooks. While CRDs also support server-side validation using Common Expression Language (CEL) for declarative validation and ValidatingAdmissionPolicies without the need for webhooks, a custom API server allows for more complex and tailored validation logic if needed.
Kubernetes allows you to serve multiple API versions for each resource type, traditionally
v1alpha1
,v1beta1
andv1
. Only one version can be specified as the storage version. All requests to other versions must be automatically converted to the version specified as storage version. With CRDs, this mechanism is implemented using conversion webhooks. Whereas in an extension API server, you can implement your own conversion mechanism, choose to mix up different storage versions (one object might be serialized asv1
, another asv2
), or rely on an external backing API. -
Directly implementing the Kubernetes API lets you format table output however you like and doesn't force you to follow the
additionalPrinterColumns
logic in CRDs. Instead, you can write your own formatter that formats the table output and custom fields in it. For example, when usingadditionalPrinterColumns
, you can display field values only following the JSONPath logic. In your own API server, you can generate and insert values on the fly, formatting the table output as you wish.
Dynamic resource registration
- The resources served by an extension api-server don't need to be pre-registered as CRDs. Once your extension API server is registered using an APIService, Kubernetes starts polling it to discover APIs and resources it can serve. After receiving a discovery response, the Kubernetes API server automatically registers all available types for this API group. Although this isn't considered common practice, you can implement logic that dynamically registers the resource types you need in your Kubernetes cluster.
When not to use the API Aggregation Layer
There are some anti-patterns where using the API Aggregation Layer isn't recommended. Let's go through them.
Unstable backend
If your API server stops responding for some reason due to an unavailable backend or other issues it may block some Kubernetes functionality. For example, when deleting namespaces, Kubernetes will wait for a response from your API server to see if there are any remaining resources. If the response doesn't come, the namespace deletion will be blocked.
Also, you might have encountered a situation where, when the metrics-server is unavailable, an extra message appears in stderr after every API request (even unrelated to metrics) stating that metrics.k8s.io
is unavailable. This is another example of how using the API Aggregation Layer can lead to problems when the api-server handling requests is unavailable.
Slow requests
If you can't guarantee an instant response for user requests, it's better to consider using a CustomResourceDefinition and controller. Otherwise, you might make your cluster less stable. Many projects implement an extension API server only for a limited set of resources, particularly for imperative logic and subresources. This recommendation is also mentioned in the official Kubernetes documentation.
Why we needed it in Cozystack
As a reminder, we're developing the open-source PaaS platform Cozystack, which can also be used as a framework for building your own private cloud. Therefore, the ability to easily extend the platform is crucial for us.
Cozystack is built on top of FluxCD. Any application is packaged into its own Helm chart, ready for deployment in a tenant namespace. Deploying any application on the platform is done by creating a HelmRelease resource, specifying the chart name and parameters for the application. All the rest logic is handled by FluxCD. This pattern allows us to easily extend the platform with new applications and provide the ability to create new applications that just need to be packaged into the appropriate Helm chart.
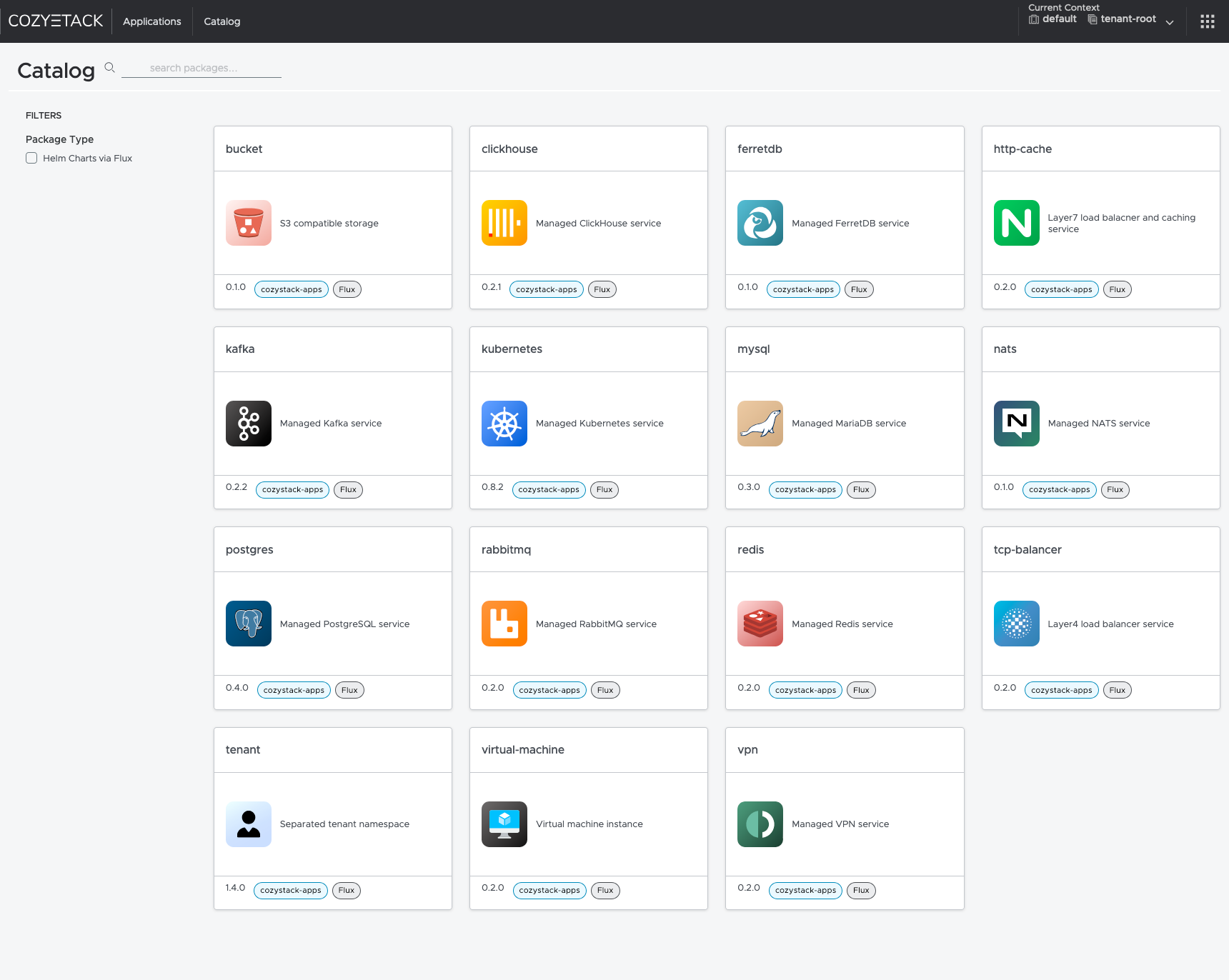
Interface of the Cozystack platform
So, in our platform, everything is configured as HelmRelease resources. However, we ran into two problems: limitations of the RBAC model and the need for a public API. Let's delve into these
Limitations of the RBAC model
The widely-deployed RBAC system in Kubernetes doesn't allow you to restrict access to a list of resources of the same kind based on labels or specific fields in the spec. When creating a role, you can limit access across the resources in the same kind only by specifying specific resource names in resourceNames
. For verbs like get or update it will work. However, filtering by resourceNames
using list verb doesn't work like that. Thus you can limit listing certain resources by kind but not by name.
- Kubernetes has a special API used to provide users with information about their permissions. This is implemented using the SelfSubjectAccessReview API. One unusual detail of these resources is that you can't view them using get or list verbs. You can only create them (using the create verb) and receive output with information about what you have access to at that moment.
So, we decided to introduce new resource types based on the names of the Helm charts they use and generate the list of available kinds dynamically at runtime in our extension api-server. This way, we can reuse Kubernetes standard RBAC model to manage access to specific resource types.
Need for a public API
Since our platform provides capabilities for deploying various managed services, we want to organize public access to the platform's API. However, we can't allow users to interact directly with resources like HelmRelease because that would let them specify arbitrary names and parameters for Helm charts to deploy, potentially compromising our system.
We wanted to give users the ability to deploy a specific service simply by creating the resource with corresponding kind in Kubernetes. The type of this resource should be named the same as the chart from which it's deployed. Here are some examples:
kind: Kubernetes
→chart: kubernetes
kind: Postgres
→chart: postgres
kind: Redis
→chart: redis
kind: VirtualMachine
→chart: virtual-machine
Moreover, we don't want to have to add a new type to codegen and recompile our extension API server every time we add a new chart for it to start being served. The schema update should be done dynamically or provided via a ConfigMap by the administrator.
Two-Way conversion
Currently, we already have integrations and a dashboard that continue to use HelmRelease resources. At this stage, we didn't want to lose the ability to support this API. Considering that we're simply translating one resource into another, support is maintained and it works both ways. If you create a HelmRelease, you'll get a custom resource in Kubernetes, and if you create a custom resource in Kubernetes, it will also be available as a HelmRelease.
We don't have any additional controllers that synchronize state between these resources. All requests to resources in our extension API server are transparently proxied to HelmRelease and vice versa. This eliminates intermediate states and the need to write controllers and synchronization logic.
Implementation
To implement the Aggregation API, you might consider starting with the following projects:
- apiserver-builder: Currently in alpha and hasn't been updated for two years. It works like kubebuilder, providing a framework for creating an extension API server, allowing you to sequentially create a project structure and generate code for your resources.
- sample-apiserver: A ready-made example of an implemented API server, based on official Kubernetes libraries, which you can use as a foundation for your project.
For practical reasons, we chose the second project. Here's what we needed to do:
Disable etcd support
In our case, we don't need it since all resources are stored directly in the Kubernetes API.
You can disable etcd options by passing nil to RecommendedOptions.Etcd
:
Generate a common resource kind
We called it Application, and it looks like this:
This is a generic type used for any application type, and its handling logic is the same for all charts.
Configure configuration loading
Since we want to configure our extension api-server via a config file, we formed the config structure in Go:
We also modified the resource registration logic so that the resources we create are registered in scheme with different Kind
values:
As a result, we got a config where you can pass all possible types and specify what they should map to:
Implement our own registry
To store state not in etcd but translate it directly into Kubernetes HelmRelease resources (and vice versa), we wrote conversion functions from Application to HelmRelease and from HelmRelease to Application:
We implemented logic to filter resources by chart name, sourceRef
, and prefix in the HelmRelease name:
Then, using this logic, we implemented the methods Get()
, Delete()
, List()
, Create()
.
You can see the full example here:
At the end of each method, we set the correct Kind
and return an unstructured.Unstructured{}
object so that Kubernetes serializes the object correctly. Otherwise, it would always serialize them with kind: Application
, which we don't want.
What did we achieve?
In Cozystack, all our types from the ConfigMap are now available in Kubernetes as-is:
kubectl api-resources | grep cozystack
buckets apps.cozystack.io/v1alpha1 true Bucket
clickhouses apps.cozystack.io/v1alpha1 true ClickHouse
etcds apps.cozystack.io/v1alpha1 true Etcd
ferretdb apps.cozystack.io/v1alpha1 true FerretDB
httpcaches apps.cozystack.io/v1alpha1 true HTTPCache
ingresses apps.cozystack.io/v1alpha1 true Ingress
kafkas apps.cozystack.io/v1alpha1 true Kafka
kuberneteses apps.cozystack.io/v1alpha1 true Kubernetes
monitorings apps.cozystack.io/v1alpha1 true Monitoring
mysqls apps.cozystack.io/v1alpha1 true MySQL
natses apps.cozystack.io/v1alpha1 true NATS
postgreses apps.cozystack.io/v1alpha1 true Postgres
rabbitmqs apps.cozystack.io/v1alpha1 true RabbitMQ
redises apps.cozystack.io/v1alpha1 true Redis
seaweedfses apps.cozystack.io/v1alpha1 true SeaweedFS
tcpbalancers apps.cozystack.io/v1alpha1 true TCPBalancer
tenants apps.cozystack.io/v1alpha1 true Tenant
virtualmachines apps.cozystack.io/v1alpha1 true VirtualMachine
vmdisks apps.cozystack.io/v1alpha1 true VMDisk
vminstances apps.cozystack.io/v1alpha1 true VMInstance
vpns apps.cozystack.io/v1alpha1 true VPN
We can work with them just like regular Kubernetes resources.
Listing S3 Buckets:
kubectl get buckets.apps.cozystack.io -n tenant-kvaps
Example output:
NAME READY AGE VERSION
foo True 22h 0.1.0
testaasd True 27h 0.1.0
Listing Kubernetes Clusters:
kubectl get kuberneteses.apps.cozystack.io -n tenant-kvaps
Example output:
NAME READY AGE VERSION
abc False 19h 0.14.0
asdte True 22h 0.13.0
Listing Virtual Machine Disks:
kubectl get vmdisks.apps.cozystack.io -n tenant-kvaps
Example output:
NAME READY AGE VERSION
docker True 21d 0.1.0
test True 18d 0.1.0
win2k25-iso True 21d 0.1.0
win2k25-system True 21d 0.1.0
Listing Virtual Machine Instances:
kubectl get vminstances.apps.cozystack.io -n tenant-kvaps
Example output:
NAME READY AGE VERSION
docker True 21d 0.1.0
test True 18d 0.1.0
win2k25 True 20d 0.1.0
We can create, modify, and delete each of them, and any interaction with them will be translated into HelmRelease resources, while also applying the resource structure and prefix in the name.
To see all related Helm releases:
kubectl get helmreleases -n tenant-kvaps -l cozystack.io/ui
Example output:
NAME AGE READY
bucket-foo 22h True
bucket-testaasd 27h True
kubernetes-abc 19h False
kubernetes-asdte 22h True
redis-test 18d True
redis-yttt 12d True
vm-disk-docker 21d True
vm-disk-test 18d True
vm-disk-win2k25-iso 21d True
vm-disk-win2k25-system 21d True
vm-instance-docker 21d True
vm-instance-test 18d True
vm-instance-win2k25 20d True
Next Steps
We don't intend to stop here with our API. In the future, we plan to add new features:
- Add validation based on an OpenAPI spec generated directly from Helm charts.
- Develop a controller that collects release notes from deployed releases and shows users access information for specific services.
- Revamp our dashboard to work directly with the new API.
Conclusion
The API Aggregation Layer allowed us to quickly and efficiently solve our problem by providing a flexible mechanism for extending the Kubernetes API with dynamically registered resources and converting them on the fly. Ultimately, this made our platform even more flexible and extensible without the need to write code for each new resource.
You can test the API yourself in the open-source PaaS platform Cozystack, starting from version v0.18.
21 Nov 2024 12:00am GMT
08 Nov 2024
Kubernetes Blog
Kubernetes v1.32 sneak peek
As we get closer to the release date for Kubernetes v1.32, the project develops and matures. Features may be deprecated, removed, or replaced with better ones for the project's overall health.
This blog outlines some of the planned changes for the Kubernetes v1.32 release, that the release team feels you should be aware of, for the continued maintenance of your Kubernetes environment and keeping up to date with the latest changes. Information listed below is based on the current status of the v1.32 release and may change before the actual release date.
The Kubernetes API removal and deprecation process
The Kubernetes project has a well-documented deprecation policy for features. This policy states that stable APIs may only be deprecated when a newer, stable version of that API is available and that APIs have a minimum lifetime for each stability level. A deprecated API has been marked for removal in a future Kubernetes release will continue to function until removal (at least one year from the deprecation). Its usage will result in a warning being displayed. Removed APIs are no longer available in the current version, so you must migrate to use the replacement instead.
-
Generally available (GA) or stable API versions may be marked as deprecated but must not be removed within a major version of Kubernetes.
-
Beta or pre-release API versions must be supported for 3 releases after the deprecation.
-
Alpha or experimental API versions may be removed in any release without prior deprecation notice; this process can become a withdrawal in cases where a different implementation for the same feature is already in place.
Whether an API is removed due to a feature graduating from beta to stable or because that API did not succeed, all removals comply with this deprecation policy. Whenever an API is removed, migration options are communicated in the deprecation guide.
Note on the withdrawal of the old DRA implementation
The enhancement #3063 introduced Dynamic Resource Allocation (DRA) in Kubernetes 1.26.
However, in Kubernetes v1.32, this approach to DRA will be significantly changed. Code related to the original implementation will be removed, leaving KEP #4381 as the "new" base functionality.
The decision to change the existing approach originated from its incompatibility with cluster autoscaling as resource availability was non-transparent, complicating decision-making for both Cluster Autoscaler and controllers. The newly added Structured Parameter model substitutes the functionality.
This removal will allow Kubernetes to handle new hardware requirements and resource claims more predictably, bypassing the complexities of back and forth API calls to the kube-apiserver.
Please also see the enhancement issue #3063 to find out more.
API removal
There is only a single API removal planned for Kubernetes v1.32:
- The
flowcontrol.apiserver.k8s.io/v1beta3
API version of FlowSchema and PriorityLevelConfiguration has been removed. To prepare for this, you can edit your existing manifests and rewrite client software to use theflowcontrol.apiserver.k8s.io/v1 API
version, available since v1.29. All existing persisted objects are accessible via the new API. Notable changes inflowcontrol.apiserver.k8s.io/v1beta3
include that the PriorityLevelConfigurationspec.limited.nominalConcurrencyShares
field only defaults to 30 when unspecified, and an explicit value of 0 is not changed to 30.
For more information, please refer to the API deprecation guide.
Sneak peek of Kubernetes v1.32
The following list of enhancements is likely to be included in the v1.32 release. This is not a commitment and the release content is subject to change.
Even more DRA enhancements!
In this release, like the previous one, the Kubernetes project continues proposing a number of enhancements to the Dynamic Resource Allocation (DRA), a key component of the Kubernetes resource management system. These enhancements aim to improve the flexibility and efficiency of resource allocation for workloads that require specialized hardware, such as GPUs, FPGAs and network adapters. This release introduces improvements, including the addition of resource health status in the Pod status, as outlined in KEP #4680.
Add resource health status to the Pod status
It isn't easy to know when a Pod uses a device that has failed or is temporarily unhealthy. KEP #4680 proposes exposing device health via Pod status
, making troubleshooting of Pod crashes easier.
Windows strikes back!
KEP #4802 adds support for graceful shutdowns of Windows nodes in Kubernetes clusters. Before this release, Kubernetes provided graceful node shutdown functionality for Linux nodes but lacked equivalent support for Windows. This enhancement enables the kubelet on Windows nodes to handle system shutdown events properly. Doing so, it ensures that Pods running on Windows nodes are gracefully terminated, allowing workloads to be rescheduled without disruption. This improvement enhances the reliability and stability of clusters that include Windows nodes, especially during a planned maintenance or any system updates.
Allow special characters in environment variables
With the graduation of this enhancement to beta, Kubernetes now allows almost all printable ASCII characters (excluding "=") to be used as environment variable names. This change addresses the limitations previously imposed on variable naming, facilitating a broader adoption of Kubernetes by accommodating various application needs. The relaxed validation will be enabled by default via the RelaxedEnvironmentVariableValidation
feature gate, ensuring that users can easily utilize environment variables without strict constraints, enhancing flexibility for developers working with applications like .NET Core that require special characters in their configurations.
Make Kubernetes aware of the LoadBalancer behavior
KEP #1860 graduates to GA, introducing the ipMode
field for a Service of type: LoadBalancer
, which can be set to either "VIP"
or "Proxy"
. This enhancement is aimed at improving how cloud providers load balancers interact with kube-proxy and it is a change transparent to the end user. The existing behavior of kube-proxy is preserved when using "VIP"
, where kube-proxy handles the load balancing. Using "Proxy"
results in traffic sent directly to the load balancer, providing cloud providers greater control over relying on kube-proxy; this means that you could see an improvement in the performance of your load balancer for some cloud providers.
Retry generate name for resources
This enhancement improves how name conflicts are handled for Kubernetes resources created with the generateName
field. Previously, if a name conflict occurred, the API server returned a 409 HTTP Conflict error and clients had to manually retry the request. With this update, the API server automatically retries generating a new name up to seven times in case of a conflict. This significantly reduces the chances of collision, ensuring smooth generation of up to 1 million names with less than a 0.1% probability of a conflict, providing more resilience for large-scale workloads.
Want to know more?
New features and deprecations are also announced in the Kubernetes release notes. We will formally announce what's new in Kubernetes v1.32 as part of the CHANGELOG for this release.
You can see the announcements of changes in the release notes for:
08 Nov 2024 12:00am GMT
28 Oct 2024
Kubernetes Blog
Spotlight on Kubernetes Upstream Training in Japan
We are organizers of Kubernetes Upstream Training in Japan. Our team is composed of members who actively contribute to Kubernetes, including individuals who hold roles such as member, reviewer, approver, and chair.
Our goal is to increase the number of Kubernetes contributors and foster the growth of the community. While Kubernetes community is friendly and collaborative, newcomers may find the first step of contributing to be a bit challenging. Our training program aims to lower that barrier and create an environment where even beginners can participate smoothly.
What is Kubernetes upstream training in Japan?
Our training started in 2019 and is held 1 to 2 times a year. Initially, Kubernetes Upstream Training was conducted as a co-located event of KubeCon (Kubernetes Contributor Summit), but we launched Kubernetes Upstream Training in Japan with the aim of increasing Japanese contributors by hosting a similar event in Japan.
Before the pandemic, the training was held in person, but since 2020, it has been conducted online. The training offers the following content for those who have not yet contributed to Kubernetes:
- Introduction to Kubernetes community
- Overview of Kubernetes codebase and how to create your first PR
- Tips and encouragement to lower participation barriers, such as language
- How to set up the development environment
- Hands-on session using kubernetes-sigs/contributor-playground
At the beginning of the program, we explain why contributing to Kubernetes is important and who can contribute. We emphasize that contributing to Kubernetes allows you to make a global impact and that Kubernetes community is looking forward to your contributions!
We also explain Kubernetes community, SIGs, and Working Groups. Next, we explain the roles and responsibilities of Member, Reviewer, Approver, Tech Lead, and Chair. Additionally, we introduce the communication tools we primarily use, such as Slack, GitHub, and mailing lists. Some Japanese speakers may feel that communicating in English is a barrier. Additionally, those who are new to the community need to understand where and how communication takes place. We emphasize the importance of taking that first step, which is the most important aspect we focus on in our training!
We then go over the structure of Kubernetes codebase, the main repositories, how to create a PR, and the CI/CD process using Prow. We explain in detail the process from creating a PR to getting it merged.
After several lectures, participants get to experience hands-on work using kubernetes-sigs/contributor-playground, where they can create a simple PR. The goal is for participants to get a feel for the process of contributing to Kubernetes.
At the end of the program, we also provide a detailed explanation of setting up the development environment for contributing to the kubernetes/kubernetes
repository, including building code locally, running tests efficiently, and setting up clusters.
Interview with participants
We conducted interviews with those who participated in our training program. We asked them about their reasons for joining, their impressions, and their future goals.
Keita Mochizuki (NTT DATA Group Corporation)
Keita Mochizuki is a contributor who consistently contributes to Kubernetes and related projects. Keita is also a professional in container security and has recently published a book. Additionally, he has made available a Roadmap for New Contributors, which is highly beneficial for those new to contributing.
Junya: Why did you decide to participate in Kubernetes Upstream Training?
Keita: Actually, I participated twice, in 2020 and 2022. In 2020, I had just started learning about Kubernetes and wanted to try getting involved in activities outside of work, so I signed up after seeing the event on Twitter by chance. However, I didn't have much knowledge at the time, and contributing to OSS felt like something beyond my reach. As a result, my understanding after the training was shallow, and I left with more of a "hmm, okay" feeling.
In 2022, I participated again when I was at a stage where I was seriously considering starting contributions. This time, I did prior research and was able to resolve my questions during the lectures, making it a very productive experience.
Junya: How did you feel after participating?
Keita: I felt that the significance of this training greatly depends on the participant's mindset. The training itself consists of general explanations and simple hands-on exercises, but it doesn't mean that attending the training will immediately lead to contributions.
Junya: What is your purpose for contributing?
Keita: My initial motivation was to "gain a deep understanding of Kubernetes and build a track record," meaning "contributing itself was the goal." Nowadays, I also contribute to address bugs or constraints I discover during my work. Additionally, through contributing, I've become less hesitant to analyze undocumented features directly from the source code.
Junya: What has been challenging about contributing?
Keita: The most difficult part was taking the first step. Contributing to OSS requires a certain level of knowledge, and leveraging resources like this training and support from others was essential. One phrase that stuck with me was, "Once you take the first step, it becomes easier to move forward." Also, in terms of continuing contributions as part of my job, the most challenging aspect is presenting the outcomes as achievements. To keep contributing over time, it's important to align it with business goals and strategies, but upstream contributions don't always lead to immediate results that can be directly tied to performance. Therefore, it's crucial to ensure mutual understanding with managers and gain their support.
Junya: What are your future goals?
Keita: My goal is to contribute to areas with a larger impact. So far, I've mainly contributed by fixing smaller bugs as my primary focus was building a track record, but moving forward, I'd like to challenge myself with contributions that have a greater impact on Kubernetes users or that address issues related to my work. Recently, I've also been working on reflecting the changes I've made to the codebase into the official documentation, and I see this as a step toward achieving my goals.
Junya: Thank you very much!
Yoshiki Fujikane (CyberAgent, Inc.)
Yoshiki Fujikane is one of the maintainers of PipeCD, a CNCF Sandbox project. In addition to developing new features for Kubernetes support in PipeCD, Yoshiki actively participates in community management and speaks at various technical conferences.
Junya: Why did you decide to participate in the Kubernetes Upstream Training?
Yoshiki: At the time I participated, I was still a student. I had only briefly worked with EKS, but I thought Kubernetes seemed complex yet cool, and I was casually interested in it. Back then, OSS felt like something out of reach, and upstream development for Kubernetes seemed incredibly daunting. While I had always been interested in OSS, I didn't know where to start. It was during this time that I learned about the Kubernetes Upstream Training and decided to take the challenge of contributing to Kubernetes.
Junya: What were your impressions after participating?
Yoshiki: I found it extremely valuable as a way to understand what it's like to be part of an OSS community. At the time, my English skills weren't very strong, so accessing primary sources of information felt like a big hurdle for me. Kubernetes is a very large project, and I didn't have a clear understanding of the overall structure, let alone what was necessary for contributing. The upstream training provided a Japanese explanation of the community structure and allowed me to gain hands-on experience with actual contributions. Thanks to the guidance I received, I was able to learn how to approach primary sources and use them as entry points for further investigation, which was incredibly helpful. This experience made me realize the importance of organizing and reviewing primary sources, and now I often dive into GitHub issues and documentation when something piques my interest. As a result, while I am no longer contributing to Kubernetes itself, the experience has been a great foundation for contributing to other projects.
Junya: What areas are you currently contributing to, and what are the other projects you're involved in?
Yoshiki: Right now, I'm no longer working with Kubernetes, but instead, I'm a maintainer of PipeCD, a CNCF Sandbox project. PipeCD is a CD tool that supports GitOps-style deployments for various application platforms. The tool originally started as an internal project at CyberAgent. With different teams adopting different platforms, PipeCD was developed to provide a unified CD platform with a consistent user experience. Currently, it supports Kubernetes, AWS ECS, Lambda, Cloud Run, and Terraform.
Junya: What role do you play within the PipeCD team?
Yoshiki: I work full-time on improving and developing Kubernetes-related features within the team. Since we provide PipeCD as a SaaS internally, my main focus is on adding new features and improving existing ones as part of that support. In addition to code contributions, I also contribute by giving talks at various events and managing community meetings to help grow the PipeCD community.
Junya: Could you explain what kind of improvements or developments you are working on with regards to Kubernetes?
Yoshiki: PipeCD supports GitOps and Progressive Delivery for Kubernetes, so I'm involved in the development of those features. Recently, I've been working on features that streamline deployments across multiple clusters.
Junya: Have you encountered any challenges while contributing to OSS?
Yoshiki: One challenge is developing features that maintain generality while meeting user use cases. When we receive feature requests while operating the internal SaaS, we first consider adding features to solve those issues. At the same time, we want PipeCD to be used by a broader audience as an OSS tool. So, I always think about whether a feature designed for one use case could be applied to another, ensuring the software remains flexible and widely usable.
Junya: What are your goals moving forward?
Yoshiki: I want to focus on expanding PipeCD's functionality. Currently, we are developing PipeCD under the slogan "One CD for All." As I mentioned earlier, it supports Kubernetes, AWS ECS, Lambda, Cloud Run, and Terraform, but there are many other platforms out there, and new platforms may emerge in the future. For this reason, we are currently developing a plugin system that will allow users to extend PipeCD on their own, and I want to push this effort forward. I'm also working on features for multi-cluster deployments in Kubernetes, and I aim to continue making impactful contributions.
Junya: Thank you very much!
Future of Kubernetes upstream training
We plan to continue hosting Kubernetes Upstream Training in Japan and look forward to welcoming many new contributors. Our next session is scheduled to take place at the end of November during CloudNative Days Winter 2024.
Moreover, our goal is to expand these training programs not only in Japan but also around the world. Kubernetes celebrated its 10th anniversary this year, and for the community to become even more active, it's crucial for people across the globe to continue contributing. While Upstream Training is already held in several regions, we aim to bring it to even more places.
We hope that as more people join Kubernetes community and contribute, our community will become even more vibrant!
28 Oct 2024 12:00am GMT
02 Oct 2024
Kubernetes Blog
Announcing the 2024 Steering Committee Election Results
The 2024 Steering Committee Election is now complete. The Kubernetes Steering Committee consists of 7 seats, 3 of which were up for election in 2024. Incoming committee members serve a term of 2 years, and all members are elected by the Kubernetes Community.
This community body is significant since it oversees the governance of the entire Kubernetes project. With that great power comes great responsibility. You can learn more about the steering committee's role in their charter.
Thank you to everyone who voted in the election; your participation helps support the community's continued health and success.
Results
Congratulations to the elected committee members whose two year terms begin immediately (listed in alphabetical order by GitHub handle):
- Antonio Ojea (@aojea), Google
- Benjamin Elder (@BenTheElder), Google
- Sascha Grunert (@saschagrunert), Red Hat
They join continuing members:
- Stephen Augustus (@justaugustus), Cisco
- Paco Xu 徐俊杰 (@pacoxu), DaoCloud
- Patrick Ohly (@pohly), Intel
- Maciej Szulik (@soltysh), Defense Unicorns
Benjamin Elder is a returning Steering Committee Member.
Big thanks!
Thank you and congratulations on a successful election to this round's election officers:
- Bridget Kromhout (@bridgetkromhout)
- Christoph Blecker (@cblecker)
- Priyanka Saggu (@Priyankasaggu11929)
Thanks to the Emeritus Steering Committee Members. Your service is appreciated by the community:
- Bob Killen (@mrbobbytables)
- Nabarun Pal (@palnabarun)
And thank you to all the candidates who came forward to run for election.
Get involved with the Steering Committee
This governing body, like all of Kubernetes, is open to all. You can follow along with Steering Committee meeting notes and weigh in by filing an issue or creating a PR against their repo. They have an open meeting on the first Monday at 8am PT of every month. They can also be contacted at their public mailing list steering@kubernetes.io.
You can see what the Steering Committee meetings are all about by watching past meetings on the YouTube Playlist.
If you want to meet some of the newly elected Steering Committee members, join us for the Steering AMA at the Kubernetes Contributor Summit North America 2024 in Salt Lake City.
This post was adapted from one written by the Contributor Comms Subproject. If you want to write stories about the Kubernetes community, learn more about us.
02 Oct 2024 8:10pm GMT
30 Sep 2024
Kubernetes Blog
Spotlight on CNCF Deaf and Hard-of-hearing Working Group (DHHWG)
In recognition of Deaf Awareness Month and the importance of inclusivity in the tech community, we are spotlighting Catherine Paganini, facilitator and one of the founding members of CNCF Deaf and Hard-of-Hearing Working Group (DHHWG). In this interview, Sandeep Kanabar, a deaf member of the DHHWG and part of the Kubernetes SIG ContribEx Communications team, sits down with Catherine to explore the impact of the DHHWG on cloud native projects like Kubernetes.
Sandeep's journey is a testament to the power of inclusion. Through his involvement in the DHHWG, he connected with members of the Kubernetes community who encouraged him to join SIG ContribEx - the group responsible for sustaining the Kubernetes contributor experience. In an ecosystem where open-source projects are actively seeking contributors and maintainers, this story highlights how important it is to create pathways for underrepresented groups, including those with disabilities, to contribute their unique perspectives and skills.
In this interview, we delve into Catherine's journey, the challenges and triumphs of establishing the DHHWG, and the vision for a more inclusive future in cloud native. We invite Kubernetes contributors, maintainers, and community members to reflect on the significance of empathy, advocacy, and community in fostering a truly inclusive environment for all, and to think about how they can support efforts to increase diversity and accessibility within their own projects.
Introduction
Sandeep Kanabar (SK): Hello Catherine, could you please introduce yourself, share your professional background, and explain your connection to the Kubernetes ecosystem?
Catherine Paganini (CP): I'm the Head of Marketing at Buoyant, the creator of Linkerd, the CNCF-graduated service mesh, and 5th CNCF project. Four years ago, I started contributing to open source. The initial motivation was to make cloud native concepts more accessible to newbies and non-technical people. Without a technical background, it was hard for me to understand what Kubernetes, containers, service meshes, etc. mean. All content was targeted at engineers already familiar with foundational concepts. Clearly, I couldn't be the only one struggling with wrapping my head around cloud native.
My first contribution was the CNCF Landscape Guide, which I co-authored with my former colleague Jason Morgan. Next, we started the CNCF Glossary, which explains cloud native concepts in simple terms. Today, the glossary has been (partially) localised into 14 languages!
Currently, I'm the co-chair of the TAG Contributor Strategy and the Facilitator of the Deaf and Hard of Hearing Working Group (DHHWG) and Blind and Visually Impaired WG (BVIWG), which is still in formation. I'm also working on a new Linux Foundation (LF) initiative called ABIDE (Accessibility and Belonging through Inclusion, Diversity, and Equity), so stay tuned to learn more about it!
Motivation and early milestones
SK: That's inspiring! Building on your passion for accessibility, what motivated you to facilitate the creation of the DHHWG? Was there a speecifc moment or experience that sparked this initiative?
CP: Last year at KubeCon Amsterdam, I learned about a great initiative by Jay Tihema that creates pathways for Maori youth into cloud native and open source. While telling my CODA (children of deaf adults) high school friend about it, I thought it'd be great to create something similar for deaf folks. A few months later, I posted about it in a LinkedIn post that the CNCF shared. Deaf people started to reach out, wanting to participate. And the rest is history.
SK: Speaking of history, since its launch, how has the DHHWG evolved? Could you highlight some of the key milestones or achievements the group has reached recently?
CP: Our WG is about a year old. It started with a few deaf engineers and me brainstorming how to make KubeCon more accessible. We published an initial draft of Best practices for an inclusive conference and shared it with the LF events team. KubeCon Chicago was two months later, and we had a couple of deaf attendees. It was the first KubeCon accessible to deaf signers. Destiny, one of our co-chairs, even participated in a keynote panel. It was incredible how quickly everything happened!
DHHWG members at KubeCon Chicago
The team has grown since then, and we've been able to do much more. With a kiosk in the project pavilion, an open space discussion, a sign language crash course, and a few media interviews, KubeCon Paris had a stronger advocacy and outreach focus. Check out this video of our team in Paris to get a glimpse of all the different KubeCon activities - it was such a great event! The team also launched the first CNCF Community Group in sign language, Deaf in Cloud Native, a glossary team that creates sign language videos for each technical term to help standardize technical signs across the globe. It's crazy to think that it all happened within one year!
Overcoming challenges and addressing misconceptions
SK: That's remarkable progress in just a year! Building such momentum must have come with its challenges. What barriers have you encountered in facilitating the DHHWG, and how did you and the group work to overcome them?
CP: The support from the community, LF, and CNCF has been incredible. The fact that we achieved so much is proof of it. The challenges are more in helping some team members overcome their fear of contributing. Most are new to open source, and it can be intimidating to put your work out there for everyone to see. The fear of being criticized in public is real; however, as they will hopefully realize over time, our community is incredibly supportive. Instead of criticizing, people tend to help improve the work, leading to better outcomes.
SK: Are there any misconceptions about the deaf and hard-of-hearing community in tech that you'd like to address?
CP: Deaf and hard of hearing individuals are very diverse - there is no one-size-fits-all. Some deaf people are oral (speak), others sign, while some lip read or prefer captions. It generally depends on how people grew up. While some people come from deaf families and sign language is their native language, others were born into hearing families who may or may not have learned how to sign. Some deaf people grew up surrounded by hearing people, while others grew up deeply embedded in Deaf culture. Hard-of-hearing individuals, on the other hand, typically can communicate well with hearing peers one-on-one in quiet settings, but loud environments or conversations with multiple people can make it hard to follow the conversation. Most rely heavily on captions. Each background and experience will shape their communication style and preferences. In short, what works for one person, doesn't necessarily work for others. So never assume and always ask about accessibility needs and preferences.
Impact and the role of allies
SK: Can you share some key impacts/outcomes of the conference best practices document?
CP: Here are the two most important ones: Captions should be on the monitor, not in an app. That's especially important during technical talks with live demos. Deaf and hard of hearing attendees will miss important information switching between captions on their phone and code on the screen.
Interpreters are most valuable during networking, not in talks (with captions). Most people come to conferences for the hallway track. That is no different for deaf attendees. If they can't network, they are missing out on key professional connections, affecting their career prospects.
SK: In your view, how crucial is the role of allies within the DHHWG, and what contributions have they made to the group's success?
CP: Deaf and hard of hearing individuals are a minority and can only do so much. Allies are the key to any diversity and inclusion initiative. As a majority, allies can help spread the word and educate their peers, playing a key role in scaling advocacy efforts. They also have the power to demand change. It's easy for companies to ignore minorities, but if the majority demands that their employers be accessible, environmentally conscious, and good citizens, they will ultimately be pushed to adapt to new societal values.
Expanding DEI efforts and future vision
SK: The importance of allies in driving change is clear. Beyond the DHHWG, are you involved in any other DEI groups or initiatives within the tech community?
CP: As mentioned above, I'm working on an initiative called ABIDE, which is still work in progress. I don't want to share too much about it yet, but what I can say is that the DHHWG will be part of it and that we just started a Blind and Visually Impaired WG (BVIWG). ABIDE will start by focusing on accessibility, so if anyone reading this has an idea for another WG, please reach out to me via the CNCF Slack @Catherine Paganini.
SK: What does the future hold for the DHHWG? Can you share details about any ongoing or upcoming initiatives?
CP: I think we've been very successful in terms of visibility and awareness so far. We can't stop, though. Awareness work is ongoing, and most people in our community haven't heard about us or met anyone on our team yet, so a lot of work still lies ahead.
DHHWG members at KubeCon Paris
The next step is to refocus on advocacy. The same thing we did with the conference best practices but for other areas. The goal is to help educate the community about what real accessibility looks like, how projects can be more accessible, and why employers should seriously consider deaf candidates while providing them with the tools they need to conduct successful interviews and employee onboarding. We need to capture all that in documents, publish it, and then get the word out. That last part is certainly the most challenging, but it's also where everyone can get involved.
Call to action
SK: Thank you for sharing your insights, Catherine. As we wrap up, do you have any final thoughts or a call to action for our readers?
CP: As we build our accessibility page, check in regularly to see what's new. Share the docs with your team, employer, and network - anyone, really. The more people understand what accessibility really means and why it matters, the more people will recognize when something isn't accessible, and be able to call out marketing-BS, which, unfortunately, is more often the case than not. We need allies to help push for change. No minority can do this on their own. So please learn about accessibility, keep an eye out for it, and call it out when something isn't accessible. We need your help!
Wrapping up
Catherine and the DHHWG's work exemplify the power of community and advocacy. As we celebrate Deaf Awareness Month, let's reflect on her role as an ally and consider how we can all contribute to building a more inclusive tech community, particularly within open-source projects like Kubernetes.
Together, we can break down barriers, challenge misconceptions, and ensure that everyone feels welcome and valued. By advocating for accessibility, supporting initiatives like the DHHWG, and fostering a culture of empathy, we can create a truly inclusive and welcoming space for all.
30 Sep 2024 12:00am GMT
24 Sep 2024
Kubernetes Blog
Spotlight on SIG Scheduling
In this SIG Scheduling spotlight we talked with Kensei Nakada, an approver in SIG Scheduling.
Introductions
Arvind: Hello, thank you for the opportunity to learn more about SIG Scheduling! Would you like to introduce yourself and tell us a bit about your role, and how you got involved with Kubernetes?
Kensei: Hi, thanks for the opportunity! I'm Kensei Nakada (@sanposhiho), a software engineer at Tetrate.io. I have been contributing to Kubernetes in my free time for more than 3 years, and now I'm an approver of SIG Scheduling in Kubernetes. Also, I'm a founder/owner of two SIG subprojects, kube-scheduler-simulator and kube-scheduler-wasm-extension.
About SIG Scheduling
AP: That's awesome! You've been involved with the project since a long time. Can you provide a brief overview of SIG Scheduling and explain its role within the Kubernetes ecosystem?
KN: As the name implies, our responsibility is to enhance scheduling within Kubernetes. Specifically, we develop the components that determine which Node is the best place for each Pod. In Kubernetes, our main focus is on maintaining the kube-scheduler, along with other scheduling-related components as part of our SIG subprojects.
AP: I see, got it! That makes me curious--what recent innovations or developments has SIG Scheduling introduced to Kubernetes scheduling?
KN: From a feature perspective, there have been several enhancements to PodTopologySpread
recently. PodTopologySpread
is a relatively new feature in the scheduler, and we are still in the process of gathering feedback and making improvements.
Most recently, we have been focusing on a new internal enhancement called QueueingHint which aims to enhance scheduling throughput. Throughput is one of our crucial metrics in scheduling. Traditionally, we have primarily focused on optimizing the latency of each scheduling cycle. QueueingHint takes a different approach, optimizing when to retry scheduling, thereby reducing the likelihood of wasting scheduling cycles.
A: That sounds interesting! Are there any other interesting topics or projects you are currently working on within SIG Scheduling?
KN: I'm leading the development of QueueingHint
which I just shared. Given that it's a big new challenge for us, we've been facing many unexpected challenges, especially around the scalability, and we're trying to solve each of them to eventually enable it by default.
And also, I believe kube-scheduler-wasm-extension (a SIG subproject) that I started last year would be interesting to many people. Kubernetes has various extensions from many components. Traditionally, extensions are provided via webhooks (extender in the scheduler) or Go SDK (Scheduling Framework in the scheduler). However, these come with drawbacks - performance issues with webhooks and the need to rebuild and replace schedulers with Go SDK, posing difficulties for those seeking to extend the scheduler but lacking familiarity with it. The project is trying to introduce a new solution to this general challenge - a WebAssembly based extension. Wasm allows users to build plugins easily, without worrying about recompiling or replacing their scheduler, and sidestepping performance concerns.
Through this project, SIG Scheduling has been learning valuable insights about WebAssembly's interaction with large Kubernetes objects. And I believe the experience that we're gaining should be useful broadly within the community, beyond SIG Scheduling.
A: Definitely! Now, there are 8 subprojects inside SIG Scheduling. Would you like to talk about them? Are there some interesting contributions by those teams you want to highlight?
KN: Let me pick up three subprojects: Kueue, KWOK and descheduler.
- Kueue
- Recently, many people have been trying to manage batch workloads with Kubernetes, and in 2022, Kubernetes community founded WG-Batch for better support for such batch workloads in Kubernetes. Kueue is a project that takes a crucial role for it. It's a job queueing controller, deciding when a job should wait, when a job should be admitted to start, and when a job should be preempted. Kueue aims to be installed on a vanilla Kubernetes cluster while cooperating with existing matured controllers (scheduler, cluster-autoscaler, kube-controller-manager, etc).
- KWOK
- KWOK is a component in which you can create a cluster of thousands of Nodes in seconds. It's mostly useful for simulation/testing as a lightweight cluster, and actually another SIG sub project kube-scheduler-simulator uses KWOK background.
- descheduler
- Descheduler is a component recreating pods that are running on undesired Nodes. In Kubernetes, scheduling constraints (
PodAffinity
,NodeAffinity
,PodTopologySpread
, etc) are honored only at Pod schedule, but it's not guaranteed that the contrtaints are kept being satisfied afterwards. Descheduler evicts Pods violating their scheduling constraints (or other undesired conditions) so that they're recreated and rescheduled. - Descheduling Framework
- One very interesting on-going project, similar to Scheduling Framework in the scheduler, aiming to make descheduling logic extensible and allow maintainers to focus on building a core engine of descheduler.
AP: Thank you for letting us know! And I have to ask, what are some of your favorite things about this SIG?
KN: What I really like about this SIG is how actively engaged everyone is. We come from various companies and industries, bringing diverse perspectives to the table. Instead of these differences causing division, they actually generate a wealth of opinions. Each view is respected, and this makes our discussions both rich and productive.
I really appreciate this collaborative atmosphere, and I believe it has been key to continuously improving our components over the years.
Contributing to SIG Scheduling
AP: Kubernetes is a community-driven project. Any recommendations for new contributors or beginners looking to get involved and contribute to SIG scheduling? Where should they start?
KN: Let me start with a general recommendation for contributing to any SIG: a common approach is to look for good-first-issue. However, you'll soon realize that many people worldwide are trying to contribute to the Kubernetes repository.
I suggest starting by examining the implementation of a component that interests you. If you have any questions about it, ask in the corresponding Slack channel (e.g., #sig-scheduling for the scheduler, #sig-node for kubelet, etc). Once you have a rough understanding of the implementation, look at issues within the SIG (e.g., sig-scheduling), where you'll find more unassigned issues compared to good-first-issue ones. You may also want to filter issues with the kind/cleanup label, which often indicates lower-priority tasks and can be starting points.
Specifically for SIG Scheduling, you should first understand the Scheduling Framework, which is the fundamental architecture of kube-scheduler. Most of the implementation is found in pkg/scheduler. I suggest starting with ScheduleOne function and then exploring deeper from there.
Additionally, apart from the main kubernetes/kubernetes repository, consider looking into sub-projects. These typically have fewer maintainers and offer more opportunities to make a significant impact. Despite being called "sub" projects, many have a large number of users and a considerable impact on the community.
And last but not least, remember contributing to the community isn't just about code. While I talked a lot about the implementation contribution, there are many ways to contribute, and each one is valuable. One comment to an issue, one feedback to an existing feature, one review comment in PR, one clarification on the documentation; every small contribution helps drive the Kubernetes ecosystem forward.
AP: Those are some pretty useful tips! And if I may ask, how do you assist new contributors in getting started, and what skills are contributors likely to learn by participating in SIG Scheduling?
KN: Our maintainers are available to answer your questions in the #sig-scheduling Slack channel. By participating, you'll gain a deeper understanding of Kubernetes scheduling and have the opportunity to collaborate and network with maintainers from diverse backgrounds. You'll learn not just how to write code, but also how to maintain a large project, design and discuss new features, address bugs, and much more.
Future Directions
AP: What are some Kubernetes-specific challenges in terms of scheduling? Are there any particular pain points?
KN: Scheduling in Kubernetes can be quite challenging because of the diverse needs of different organizations with different business requirements. Supporting all possible use cases in kube-scheduler is impossible. Therefore, extensibility is a key focus for us. A few years ago, we rearchitected kube-scheduler with Scheduling Framework, which offers flexible extensibility for users to implement various scheduling needs through plugins. This allows maintainers to focus on the core scheduling features and the framework runtime.
Another major issue is maintaining sufficient scheduling throughput. Typically, a Kubernetes cluster has only one kube-scheduler, so its throughput directly affects the overall scheduling scalability and, consequently, the cluster's scalability. Although we have an internal performance test (scheduler_perf), unfortunately, we sometimes overlook performance degradation in less common scenarios. It's difficult as even small changes, which look irrelevant to performance, can lead to degradation.
AP: What are some upcoming goals or initiatives for SIG Scheduling? How do you envision the SIG evolving in the future?
KN: Our primary goal is always to build and maintain extensible and stable scheduling runtime, and I bet this goal will remain unchanged forever.
As already mentioned, extensibility is key to solving the challenge of the diverse needs of scheduling. Rather than trying to support every different use case directly in kube-scheduler, we will continue to focus on enhancing extensibility so that it can accommodate various use cases. kube-scheduler-wasm-extension that I mentioned is also part of this initiative.
Regarding stability, introducing new optimizations like QueueHint is one of our strategies. Additionally, maintaining throughput is also a crucial goal towards the future. We're planning to enhance our throughput monitoring (ref), so that we can notice degradation as much as possible on our own before releasing. But, realistically, we can't cover every possible scenario. We highly appreciate any attention the community can give to scheduling throughput and encourage feedback and alerts regarding performance issues!
Closing Remarks
AP: Finally, what message would you like to convey to those who are interested in learning more about SIG Scheduling?
KN: Scheduling is one of the most complicated areas in Kubernetes, and you may find it difficult at first. But, as I shared earlier, you can find many opportunities for contributions, and many maintainers are willing to help you understand things. We know your unique perspective and skills are what makes our open source so powerful 😊
Feel free to reach out to us in Slack (#sig-scheduling) or meetings. I hope this article interests everyone and we can see new contributors!
AP: Thank you so much for taking the time to do this! I'm confident that many will find this information invaluable for understanding more about SIG Scheduling and for contributing to the SIG.
24 Sep 2024 12:00am GMT
23 Aug 2024
Kubernetes Blog
Kubernetes v1.31: kubeadm v1beta4
As part of the Kubernetes v1.31 release, kubeadm
is adopting a new (v1beta4) version of its configuration file format. Configuration in the previous v1beta3 format is now formally deprecated, which means it's supported but you should migrate to v1beta4 and stop using the deprecated format. Support for v1beta3 configuration will be removed after a minimum of 3 Kubernetes minor releases.
In this article, I'll walk you through key changes; I'll explain about the kubeadm v1beta4 configuration format, and how to migrate from v1beta3 to v1beta4.
You can read the reference for the v1beta4 configuration format: kubeadm Configuration (v1beta4).
A list of changes since v1beta3
This version improves on the v1beta3 format by fixing some minor issues and adding a few new fields.
To put it simply,
- Two new configuration elements: ResetConfiguration and UpgradeConfiguration
- For InitConfiguration and JoinConfiguration,
dryRun
mode andnodeRegistration.imagePullSerial
are supported - For ClusterConfiguration, there are new fields including
certificateValidityPeriod
,caCertificateValidityPeriod
,encryptionAlgorithm
,dns.disabled
andproxy.disabled
. - Support
extraEnvs
for all control plan components extraArgs
changed from a map to structured extra arguments for duplicates- Add a
timeouts
structure for init, join, upgrade and reset.
For details, you can see the official document below:
- Support custom environment variables in control plane components under
ClusterConfiguration
. UseapiServer.extraEnvs
,controllerManager.extraEnvs
,scheduler.extraEnvs
,etcd.local.extraEnvs
. - The ResetConfiguration API type is now supported in v1beta4. Users are able to reset a node by passing a
--config
file tokubeadm reset
. dryRun
mode is now configurable in InitConfiguration and JoinConfiguration.- Replace the existing string/string extra argument maps with structured extra arguments that support duplicates. The change applies to
ClusterConfiguration
-apiServer.extraArgs
,controllerManager.extraArgs
,scheduler.extraArgs
,etcd.local.extraArgs
. Also tonodeRegistrationOptions.kubeletExtraArgs
. - Added
ClusterConfiguration.encryptionAlgorithm
that can be used to set the asymmetric encryption algorithm used for this cluster's keys and certificates. Can be one of "RSA-2048" (default), "RSA-3072", "RSA-4096" or "ECDSA-P256". - Added
ClusterConfiguration.dns.disabled
andClusterConfiguration.proxy.disabled
that can be used to disable the CoreDNS and kube-proxy addons during cluster initialization. Skipping the related addons phases, during cluster creation will set the same fields totrue
. - Added the
nodeRegistration.imagePullSerial
field inInitConfiguration
andJoinConfiguration
, which can be used to control if kubeadm pulls images serially or in parallel. - The UpgradeConfiguration kubeadm API is now supported in v1beta4 when passing
--config
tokubeadm upgrade
subcommands. For upgrade subcommands, the usage of component configuration for kubelet and kube-proxy, as well as InitConfiguration and ClusterConfiguration, is now deprecated and will be ignored when passing--config
. - Added a
timeouts
structure toInitConfiguration
,JoinConfiguration
,ResetConfiguration
andUpgradeConfiguration
that can be used to configure various timeouts. TheClusterConfiguration.timeoutForControlPlane
field is replaced bytimeouts.controlPlaneComponentHealthCheck
. TheJoinConfiguration.discovery.timeout
is replaced bytimeouts.discovery
. - Added a
certificateValidityPeriod
andcaCertificateValidityPeriod
fields toClusterConfiguration
. These fields can be used to control the validity period of certificates generated by kubeadm during sub-commands such asinit
,join
,upgrade
andcerts
. Default values continue to be 1 year for non-CA certificates and 10 years for CA certificates. Also note that only non-CA certificates are renewable bykubeadm certs renew
.
These changes simplify the configuration of tools that use kubeadm and improve the extensibility of kubeadm itself.
How to migrate v1beta3 configuration to v1beta4?
If your configuration is not using the latest version, it is recommended that you migrate using the kubeadm config migrate command.
This command reads an existing configuration file that uses the old format, and writes a new file that uses the current format.
Example
Using kubeadm v1.31, run kubeadm config migrate --old-config old-v1beta3.yaml --new-config new-v1beta4.yaml
How do I get involved?
Huge thanks to all the contributors who helped with the design, implementation, and review of this feature:
- Lubomir I. Ivanov (neolit123)
- Dave Chen(chendave)
- Paco Xu (pacoxu)
- Sata Qiu(sataqiu)
- Baofa Fan(carlory)
- Calvin Chen(calvin0327)
- Ruquan Zhao(ruquanzhao)
For those interested in getting involved in future discussions on kubeadm configuration, you can reach out kubeadm or SIG-cluster-lifecycle by several means:
- v1beta4 related items are tracked in kubeadm issue #2890.
- Slack: #kubeadm or #sig-cluster-lifecycle
- Mailing list
23 Aug 2024 12:00am GMT